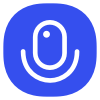
Sign up to save your podcasts
Or
This whitepaper provides a comprehensive overview of foundational large language models (LLMs) and text generation. It traces the evolution of transformer architectures, detailing key models from GPT-1 to Gemini and open-source alternatives. The authors explain training and fine-tuning methodologies, including supervised learning and reinforcement learning from human feedback, as well as parameter-efficient techniques. Furthermore, the paper discusses strategies for utilizing LLMs effectively, such as prompt engineering and sampling, and explores methods for accelerating inference to improve speed and efficiency. Finally, it highlights a wide array of applications demonstrating the transformative potential of LLMs across various domains.
This whitepaper provides a comprehensive overview of foundational large language models (LLMs) and text generation. It traces the evolution of transformer architectures, detailing key models from GPT-1 to Gemini and open-source alternatives. The authors explain training and fine-tuning methodologies, including supervised learning and reinforcement learning from human feedback, as well as parameter-efficient techniques. Furthermore, the paper discusses strategies for utilizing LLMs effectively, such as prompt engineering and sampling, and explores methods for accelerating inference to improve speed and efficiency. Finally, it highlights a wide array of applications demonstrating the transformative potential of LLMs across various domains.