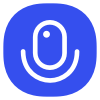
Sign up to save your podcasts
Or
In this episode, we dive into the nuts and bolts of MLOps—the crucial discipline that bridges the gap between machine learning development and real-world deployment. Drawing insights from Introducing MLOps by Mark Treveil and the Dataiku team, we explore what it really takes to operationalize machine learning in enterprise environments.
From building reproducible models and setting up robust CI/CD pipelines to managing data drift and enforcing responsible AI practices, we walk through the entire lifecycle of a model in production. You'll learn about the diverse roles that make MLOps successful, how to align governance with risk, and why monitoring and feedback loops are essential to long-term model health. With practical case studies in credit risk and marketing, this episode delivers a comprehensive roadmap for deploying ML systems that scale—safely, ethically, and efficiently.
In this episode, we dive into the nuts and bolts of MLOps—the crucial discipline that bridges the gap between machine learning development and real-world deployment. Drawing insights from Introducing MLOps by Mark Treveil and the Dataiku team, we explore what it really takes to operationalize machine learning in enterprise environments.
From building reproducible models and setting up robust CI/CD pipelines to managing data drift and enforcing responsible AI practices, we walk through the entire lifecycle of a model in production. You'll learn about the diverse roles that make MLOps successful, how to align governance with risk, and why monitoring and feedback loops are essential to long-term model health. With practical case studies in credit risk and marketing, this episode delivers a comprehensive roadmap for deploying ML systems that scale—safely, ethically, and efficiently.