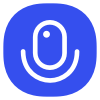
Sign up to save your podcasts
Or
https://arxiv.org/abs/2402.07630
The paper "G-Retriever" introduces a new method for question answering on textual graphs. It addresses the challenge of enabling users to interact with graphs through a conversational interface. The core innovation is a retrieval-augmented generation (RAG) approach specifically designed for textual graphs, using a Prize-Collecting Steiner Tree optimization to handle large graphs and mitigate hallucinations. A new benchmark, GraphQA, was developed to facilitate research in this area. Empirical results demonstrate that G-Retriever outperforms existing methods on various textual graph tasks. The study showcases the method's scalability and its effectiveness in reducing hallucination.
https://arxiv.org/abs/2402.07630
The paper "G-Retriever" introduces a new method for question answering on textual graphs. It addresses the challenge of enabling users to interact with graphs through a conversational interface. The core innovation is a retrieval-augmented generation (RAG) approach specifically designed for textual graphs, using a Prize-Collecting Steiner Tree optimization to handle large graphs and mitigate hallucinations. A new benchmark, GraphQA, was developed to facilitate research in this area. Empirical results demonstrate that G-Retriever outperforms existing methods on various textual graph tasks. The study showcases the method's scalability and its effectiveness in reducing hallucination.