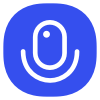
Sign up to save your podcasts
Or
This podcast will explore the world of generative models, specifically focusing on two groundbreaking architectures: Variational Autoencoders (VAEs) and Generative Adversarial Networks (GANs). VAEs, as described in source, utilise a variational approximation of the true posterior distribution to generate data, while GANs employ a minimax two-player game between a generator and a discriminator, as explained in source. We will examine how VAEs leverage directed graphical models1 and KL-divergence to approximate the intractable posterior distribution, enabling efficient data generation. Conversely, we will explore how GANs, through the adversarial training process, push the generator to produce increasingly realistic samples, ultimately aiming to match the data distribution. By comparing and contrasting these two powerful approaches, this podcast will provide insights into the fascinating world of generative modelling and its transformative impact on various fields.
This podcast will explore the world of generative models, specifically focusing on two groundbreaking architectures: Variational Autoencoders (VAEs) and Generative Adversarial Networks (GANs). VAEs, as described in source, utilise a variational approximation of the true posterior distribution to generate data, while GANs employ a minimax two-player game between a generator and a discriminator, as explained in source. We will examine how VAEs leverage directed graphical models1 and KL-divergence to approximate the intractable posterior distribution, enabling efficient data generation. Conversely, we will explore how GANs, through the adversarial training process, push the generator to produce increasingly realistic samples, ultimately aiming to match the data distribution. By comparing and contrasting these two powerful approaches, this podcast will provide insights into the fascinating world of generative modelling and its transformative impact on various fields.