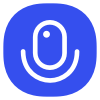
Sign up to save your podcasts
Or
This research paper proposes a novel framework for estimating generative models using adversarial nets. The core idea is to train two neural networks simultaneously: a generative model that captures the data distribution and a discriminative model that estimates the probability of a sample coming from the training data rather than the generative model. The generative model aims to fool the discriminative model, while the discriminative model strives to accurately distinguish real data from generated samples. This adversarial process leads to improved performance for both models, resulting in the generative model learning to produce highly realistic data.
Link to Paper: https://arxiv.org/abs/1406.2661
This research paper proposes a novel framework for estimating generative models using adversarial nets. The core idea is to train two neural networks simultaneously: a generative model that captures the data distribution and a discriminative model that estimates the probability of a sample coming from the training data rather than the generative model. The generative model aims to fool the discriminative model, while the discriminative model strives to accurately distinguish real data from generated samples. This adversarial process leads to improved performance for both models, resulting in the generative model learning to produce highly realistic data.
Link to Paper: https://arxiv.org/abs/1406.2661