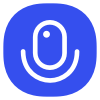
Sign up to save your podcasts
Or
This episode breaks down the research paper "GPipe: Easy Scaling with Micro-Batch Pipeline Parallelism," which proposes a new method for training very large neural networks by partitioning the model across multiple accelerators and using a novel batch-splitting pipelining algorithm. This approach allows for the efficient training of larger models than previously possible, achieving almost linear speedup with the number of accelerators.
Audio : (Spotify) https://open.spotify.com/episode/4zXyQKSdiSUFK7HkAi6pxO?si=eWWrNsURSqGtw6Phf4tpJg
Paper: https://arxiv.org/abs/1811.06965
This episode breaks down the research paper "GPipe: Easy Scaling with Micro-Batch Pipeline Parallelism," which proposes a new method for training very large neural networks by partitioning the model across multiple accelerators and using a novel batch-splitting pipelining algorithm. This approach allows for the efficient training of larger models than previously possible, achieving almost linear speedup with the number of accelerators.
Audio : (Spotify) https://open.spotify.com/episode/4zXyQKSdiSUFK7HkAi6pxO?si=eWWrNsURSqGtw6Phf4tpJg
Paper: https://arxiv.org/abs/1811.06965