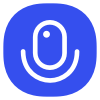
Sign up to save your podcasts
Or
This research introduces a novel method for generating counterfactual explanations for graph-based predictions.The approach utilizes a permutation equivariant graph variational autoencoder (PEGVAE) to create a meaningful latent space representation of graphs. By traversing this latent space guided by the classifier, the method identifies minimally altered graphs with a different classification. This technique addresses the challenges posed by the discrete nature and permutation invariance of graphs. Experiments on molecular datasets demonstrate the method's ability to produce robust and high-performing counterfactual explanations compared to baseline approaches. The work offers a way to understand how to change a graph to alter its predicted outcome without explicitly defining a graph distance metric.
This research introduces a novel method for generating counterfactual explanations for graph-based predictions.The approach utilizes a permutation equivariant graph variational autoencoder (PEGVAE) to create a meaningful latent space representation of graphs. By traversing this latent space guided by the classifier, the method identifies minimally altered graphs with a different classification. This technique addresses the challenges posed by the discrete nature and permutation invariance of graphs. Experiments on molecular datasets demonstrate the method's ability to produce robust and high-performing counterfactual explanations compared to baseline approaches. The work offers a way to understand how to change a graph to alter its predicted outcome without explicitly defining a graph distance metric.