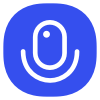
Sign up to save your podcasts
Or
This paper explores inductive biases in exchangeable sequence modeling, focusing on architectural choices and inferential methods, particularly for decision-making tasks. It highlights a limitation of single-step inference in distinguishing between epistemic and aleatoric uncertainty, advocating for multi-step inference for better uncertainty quantification and downstream performance. The authors also examine Transformer architectures designed for exchangeable sequences, revealing that existing masking schemes achieve conditional permutation invariance but do not guarantee full exchangeability, and surprisingly, they underperform standard causal models.
This paper explores inductive biases in exchangeable sequence modeling, focusing on architectural choices and inferential methods, particularly for decision-making tasks. It highlights a limitation of single-step inference in distinguishing between epistemic and aleatoric uncertainty, advocating for multi-step inference for better uncertainty quantification and downstream performance. The authors also examine Transformer architectures designed for exchangeable sequences, revealing that existing masking schemes achieve conditional permutation invariance but do not guarantee full exchangeability, and surprisingly, they underperform standard causal models.