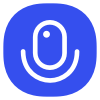
Sign up to save your podcasts
Or
For decades, manufacturers have relied on traditional analytics—correlations, trendlines, dashboards—to make operational decisions. But there's a limit:
Correlation ≠ Causation
Just because two variables move together doesn’t mean one causes the other.
This blind spot can lead to poor decisions and surface-level fixes that don’t solve the real issue.
For example, a machine’s temperature spikes often coincide with defects. Traditional analytics might alert you when it happens—but not why. Is it the temperature? A faulty sensor? Operator error?
Causal Inference flips the script. Instead of just observing data patterns, it asks:
“What actually caused this outcome?”
I recently sat down with Daniele Gamba, CEO of AISent Srl to learn more about building industrial intelligence solutions with Caussal AI.
5
11 ratings
For decades, manufacturers have relied on traditional analytics—correlations, trendlines, dashboards—to make operational decisions. But there's a limit:
Correlation ≠ Causation
Just because two variables move together doesn’t mean one causes the other.
This blind spot can lead to poor decisions and surface-level fixes that don’t solve the real issue.
For example, a machine’s temperature spikes often coincide with defects. Traditional analytics might alert you when it happens—but not why. Is it the temperature? A faulty sensor? Operator error?
Causal Inference flips the script. Instead of just observing data patterns, it asks:
“What actually caused this outcome?”
I recently sat down with Daniele Gamba, CEO of AISent Srl to learn more about building industrial intelligence solutions with Caussal AI.
7,899 Listeners
3 Listeners
98 Listeners