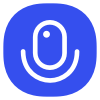
Sign up to save your podcasts
Or
Serg Masis is the author of best-selling book 'Interpretable Machine Learning with Python' and senior Data Scientist at Sygenta. He has mentored many data scientists around the world.
Timestamps:
00:00 intro
08:30 Old 4.77 MH z Computer, Late 80s and Programming
11:51 Fairness, Accountability and Transparency in Machine Learning, Startup and Harvard
16:33 Fairness vs Preciseness, Bias and Variance Tradeoff, Are Engineers to blame?
21:43 Mask-Detection Problem in Coded-Bias, Biased Samples, Surveillance using CV
32:38 Fixing Biased Datasets, Augmenting Data and Limitations
37:39 Algorithmic Optimisation and Explainability
40:51 Eric Schmidt on Behavioral Prediction, SHAP values, Tree and DeepExplainers
44:50 Challenges of using SHAP and LIME & Big Data
49:37 GPT3, Large Models and ROI on Explainability
01:00:00 TCAS, Collision Risks and Interpretability, Ransom Attacks
01:08:09 Guitar, Bass, and Led Zepplin
01:09:31 Birth Order and IQ, Science vs Folk Wisdom
01:13:30 Reverse Discrimination & Men, Bias in Child Custody, Prison Sentences, and Incarceration
01:23:11 Receidivism to Criminal Behaviour, Ethnic over-representation & Systematic Racism
01:24:44 Human Judges vs AI, Absolute Fairness, Food and Parole
01:30:20 Face Detection in China, Privacy vs Convenience, Feature Engineering and Model Parsimony
01:35:51 Sparsity, Interaction Effects, and Multicollinearity
01:38:23 Four levels of Global and Local Predictive Explainability
01:43:17 Recursive and Sequential Feature Selection
01:47:42 Ensemble, Blended and Stacked Models and Interpretability
01:53:45 In-Processing and Post-Processing Bias Mitigation
01:57:00 Future of Interpretable AI
5
33 ratings
Serg Masis is the author of best-selling book 'Interpretable Machine Learning with Python' and senior Data Scientist at Sygenta. He has mentored many data scientists around the world.
Timestamps:
00:00 intro
08:30 Old 4.77 MH z Computer, Late 80s and Programming
11:51 Fairness, Accountability and Transparency in Machine Learning, Startup and Harvard
16:33 Fairness vs Preciseness, Bias and Variance Tradeoff, Are Engineers to blame?
21:43 Mask-Detection Problem in Coded-Bias, Biased Samples, Surveillance using CV
32:38 Fixing Biased Datasets, Augmenting Data and Limitations
37:39 Algorithmic Optimisation and Explainability
40:51 Eric Schmidt on Behavioral Prediction, SHAP values, Tree and DeepExplainers
44:50 Challenges of using SHAP and LIME & Big Data
49:37 GPT3, Large Models and ROI on Explainability
01:00:00 TCAS, Collision Risks and Interpretability, Ransom Attacks
01:08:09 Guitar, Bass, and Led Zepplin
01:09:31 Birth Order and IQ, Science vs Folk Wisdom
01:13:30 Reverse Discrimination & Men, Bias in Child Custody, Prison Sentences, and Incarceration
01:23:11 Receidivism to Criminal Behaviour, Ethnic over-representation & Systematic Racism
01:24:44 Human Judges vs AI, Absolute Fairness, Food and Parole
01:30:20 Face Detection in China, Privacy vs Convenience, Feature Engineering and Model Parsimony
01:35:51 Sparsity, Interaction Effects, and Multicollinearity
01:38:23 Four levels of Global and Local Predictive Explainability
01:43:17 Recursive and Sequential Feature Selection
01:47:42 Ensemble, Blended and Stacked Models and Interpretability
01:53:45 In-Processing and Post-Processing Bias Mitigation
01:57:00 Future of Interpretable AI