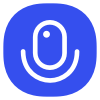
Sign up to save your podcasts
Or
Seventy3: 用NotebookLM将论文生成播客,让大家跟着AI一起进步。
今天的主题是:Were RNNs All We Needed?Main Theme:
This research paper revisits traditional recurrent neural networks (RNNs) like LSTMs and GRUs, proposing simplified versions – minLSTM and minGRU – that address the scalability limitations of their predecessors while achieving comparable performance to modern sequence models.
Key Ideas and Facts:
Conclusion:
This research challenges the current dominance of Transformers by demonstrating that minimally simplified versions of LSTMs and GRUs can achieve comparable performance with significantly improved efficiency. This opens up new possibilities for leveraging efficient recurrent models for long sequence modeling tasks.
Limitations:
Overall:
This paper presents a compelling case for reconsidering the potential of RNNs in the age of Transformers. By simplifying LSTMs and GRUs, the authors unlock efficiency gains without compromising performance, paving the way for further research and development of efficient recurrent models for long sequence modeling.
原文链接:https://arxiv.org/abs/2410.01201
Seventy3: 用NotebookLM将论文生成播客,让大家跟着AI一起进步。
今天的主题是:Were RNNs All We Needed?Main Theme:
This research paper revisits traditional recurrent neural networks (RNNs) like LSTMs and GRUs, proposing simplified versions – minLSTM and minGRU – that address the scalability limitations of their predecessors while achieving comparable performance to modern sequence models.
Key Ideas and Facts:
Conclusion:
This research challenges the current dominance of Transformers by demonstrating that minimally simplified versions of LSTMs and GRUs can achieve comparable performance with significantly improved efficiency. This opens up new possibilities for leveraging efficient recurrent models for long sequence modeling tasks.
Limitations:
Overall:
This paper presents a compelling case for reconsidering the potential of RNNs in the age of Transformers. By simplifying LSTMs and GRUs, the authors unlock efficiency gains without compromising performance, paving the way for further research and development of efficient recurrent models for long sequence modeling.
原文链接:https://arxiv.org/abs/2410.01201