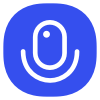
Sign up to save your podcasts
Or
Seventy3: 用NotebookLM将论文生成播客,让大家跟着AI一起进步。
今天的主题是:Differential TransformerSource: Ye, Tianzhu, et al. "Differential Transformer." arXiv preprint arXiv:2410.05258 (2024).
Main Theme: The paper introduces DIFF Transformer, a novel Transformer architecture designed to enhance the attention mechanism in Large Language Models (LLMs) by mitigating the issue of over-attention to irrelevant context.
Key Ideas & Facts:
"Transformer tends to allocate only a small proportion of attention scores to the correct answer, while disproportionately focusing on irrelevant context."
"The differential attention mechanism eliminates attention noise, encouraging models to focus on critical information. The approach is analogous to noise-canceling headphones and differential amplifiers in electrical engineering, where the difference between two signals cancels out common-mode noise."
Quotes:
Overall: DIFF Transformer presents a promising new architecture for enhancing LLMs by addressing the critical issue of attention noise. The proposed differential attention mechanism demonstrates significant potential for improving scalability, long-context understanding, task performance, and efficiency in LLMs.
原文链接:https://arxiv.org/abs/2410.05258
Seventy3: 用NotebookLM将论文生成播客,让大家跟着AI一起进步。
今天的主题是:Differential TransformerSource: Ye, Tianzhu, et al. "Differential Transformer." arXiv preprint arXiv:2410.05258 (2024).
Main Theme: The paper introduces DIFF Transformer, a novel Transformer architecture designed to enhance the attention mechanism in Large Language Models (LLMs) by mitigating the issue of over-attention to irrelevant context.
Key Ideas & Facts:
"Transformer tends to allocate only a small proportion of attention scores to the correct answer, while disproportionately focusing on irrelevant context."
"The differential attention mechanism eliminates attention noise, encouraging models to focus on critical information. The approach is analogous to noise-canceling headphones and differential amplifiers in electrical engineering, where the difference between two signals cancels out common-mode noise."
Quotes:
Overall: DIFF Transformer presents a promising new architecture for enhancing LLMs by addressing the critical issue of attention noise. The proposed differential attention mechanism demonstrates significant potential for improving scalability, long-context understanding, task performance, and efficiency in LLMs.
原文链接:https://arxiv.org/abs/2410.05258