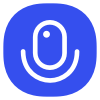
Sign up to save your podcasts
Or
Seventy3: 用NotebookLM将论文生成播客,让大家跟着AI一起进步。
今天的主题是:FAN: Fourier Analysis NetworksThis briefing document reviews the key themes and findings from the research paper "FAN: Fourier Analysis Networks". The paper tackles the challenge of modeling periodicity in neural networks, a crucial aspect often overlooked by popular architectures like MLPs and Transformers.
Key Problem: Existing neural networks excel at interpolation within the training data domain but struggle with extrapolation, especially when dealing with periodic functions. They tend to memorize periodic data instead of understanding the underlying principles of periodicity, hindering their generalization capabilities.
Proposed Solution: The paper introduces FAN (Fourier Analysis Network), a novel architecture that explicitly integrates periodicity into the network structure using Fourier Series. This addresses the limitation of data-driven optimization in traditional networks by introducing an inherent understanding of periodic patterns.
Key Features of FAN:
*"By leveraging the power of Fourier Series, we explicitly encode periodic patterns within the neural network, offering a way to model the general principles from the data." *
"FAN significantly outperforms the baselines in all these tasks of periodicity modeling...Moreover, FAN performs exceptionally well on test data both within and outside the domain of the training data, indicating that it is genuinely modeling periodicity rather than merely memorizing the training data."
"As a promising substitute to MLP, FAN improves the model’s generalization performance meanwhile reducing the number of parameters and floating point of operations (FLOPs) employed."
Experimental Results:
Future Directions:
The authors highlight the potential of scaling up FAN and exploring its application to a wider range of tasks. Further investigation into the theoretical properties and practical implications of integrating periodicity into neural networks is also encouraged.
Conclusion:
FAN presents a novel approach to address the challenge of periodicity modeling in neural networks. Its strong empirical performance across diverse tasks, coupled with its efficiency and potential for broader applications, makes it a promising advancement in the field of deep learning. FAN's success suggests that explicitly incorporating domain-specific knowledge, such as periodicity, into neural network architectures can lead to significant improvements in learning and generalization.
原文链接:https://arxiv.org/abs/2410.02675
Seventy3: 用NotebookLM将论文生成播客,让大家跟着AI一起进步。
今天的主题是:FAN: Fourier Analysis NetworksThis briefing document reviews the key themes and findings from the research paper "FAN: Fourier Analysis Networks". The paper tackles the challenge of modeling periodicity in neural networks, a crucial aspect often overlooked by popular architectures like MLPs and Transformers.
Key Problem: Existing neural networks excel at interpolation within the training data domain but struggle with extrapolation, especially when dealing with periodic functions. They tend to memorize periodic data instead of understanding the underlying principles of periodicity, hindering their generalization capabilities.
Proposed Solution: The paper introduces FAN (Fourier Analysis Network), a novel architecture that explicitly integrates periodicity into the network structure using Fourier Series. This addresses the limitation of data-driven optimization in traditional networks by introducing an inherent understanding of periodic patterns.
Key Features of FAN:
*"By leveraging the power of Fourier Series, we explicitly encode periodic patterns within the neural network, offering a way to model the general principles from the data." *
"FAN significantly outperforms the baselines in all these tasks of periodicity modeling...Moreover, FAN performs exceptionally well on test data both within and outside the domain of the training data, indicating that it is genuinely modeling periodicity rather than merely memorizing the training data."
"As a promising substitute to MLP, FAN improves the model’s generalization performance meanwhile reducing the number of parameters and floating point of operations (FLOPs) employed."
Experimental Results:
Future Directions:
The authors highlight the potential of scaling up FAN and exploring its application to a wider range of tasks. Further investigation into the theoretical properties and practical implications of integrating periodicity into neural networks is also encouraged.
Conclusion:
FAN presents a novel approach to address the challenge of periodicity modeling in neural networks. Its strong empirical performance across diverse tasks, coupled with its efficiency and potential for broader applications, makes it a promising advancement in the field of deep learning. FAN's success suggests that explicitly incorporating domain-specific knowledge, such as periodicity, into neural network architectures can lead to significant improvements in learning and generalization.
原文链接:https://arxiv.org/abs/2410.02675