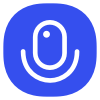
Sign up to save your podcasts
Or
This survey examines the burgeoning field of large language models (LLMs) as planning modules for autonomous agents, offering the first systematic overview of recent efforts to enhance their planning abilities. The authors categorize existing research into five key areas: task decomposition, plan selection, external module integration, reflection and refinement, and memory augmentation, providing comprehensive analysis for each. The paper further discusses challenges such as hallucinations, plan feasibility and efficiency, handling multi-modal feedback, and the need for better evaluation methods. Through experiments on interactive benchmarks, the study validates the performance of representative planning techniques, concluding with insights into future research directions aimed at overcoming current limitations and further developing the planning capabilities of LLM-based agents.
This survey examines the burgeoning field of large language models (LLMs) as planning modules for autonomous agents, offering the first systematic overview of recent efforts to enhance their planning abilities. The authors categorize existing research into five key areas: task decomposition, plan selection, external module integration, reflection and refinement, and memory augmentation, providing comprehensive analysis for each. The paper further discusses challenges such as hallucinations, plan feasibility and efficiency, handling multi-modal feedback, and the need for better evaluation methods. Through experiments on interactive benchmarks, the study validates the performance of representative planning techniques, concluding with insights into future research directions aimed at overcoming current limitations and further developing the planning capabilities of LLM-based agents.