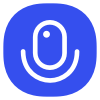
Sign up to save your podcasts
Or
Research paper: https://arxiv.org/pdf/2502.04677
Authors: Gregory Dexter, Shao Tang, Ata Fatahi Baarzi, Qingquan Song, Tejas Dharamsi, and Aman Gupta
Introduction
In this episode, we explore the challenge of efficiently deploying large language models (LLMs) in online settings, where strict latency constraints—such as time-to-first-token (TTFT) and time-per-output-token (TPOT)—must be met. As demand for AI-generated content grows, optimizing inference performance becomes a critical bottleneck.
Key Topics Covered
Conclusion
This research highlights the need for advanced scheduling strategies to improve LLM efficiency in real-world applications. Tune in to learn how k-LPM is pushing the boundaries of AI inference optimization!
Research paper: https://arxiv.org/pdf/2502.04677
Authors: Gregory Dexter, Shao Tang, Ata Fatahi Baarzi, Qingquan Song, Tejas Dharamsi, and Aman Gupta
Introduction
In this episode, we explore the challenge of efficiently deploying large language models (LLMs) in online settings, where strict latency constraints—such as time-to-first-token (TTFT) and time-per-output-token (TPOT)—must be met. As demand for AI-generated content grows, optimizing inference performance becomes a critical bottleneck.
Key Topics Covered
Conclusion
This research highlights the need for advanced scheduling strategies to improve LLM efficiency in real-world applications. Tune in to learn how k-LPM is pushing the boundaries of AI inference optimization!