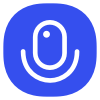
Sign up to save your podcasts
Or
In this episode we're discussing this paper:
"Large Language Models Reflect the Ideology of their Creators"
by
Maarten Buyl, Alexander Rogiers, Sander Noels, Iris Dominguez-Catena, Edith Heiter, Raphael Romero, Iman Johary, Alexandru-Cristian Mara, Jefrey Lijffijt, Tijl De Bie
https://arxiv.org/pdf/2410.18417
We will delve into a groundbreaking study exploring the ideological leanings of large language models (LLMs). Through analyzing LLMs' responses to prompts about diverse historical figures, researchers found that these models often mirror the perspectives of their creators, exhibiting biases influenced by language, regional factors, and the companies behind their development.
Join us as we discuss the implications of these findings—how LLMs may be far from ideologically neutral, the potential risks of political influence, and the pressing need for transparency and regulatory measures in AI development.
***
Ideological Stances of LLMs Across Models, Languages, and Regions
The paper explores how the ideological stance of LLMs varies based on different factors, including:
● Model Origin: LLMs created in Western and non-Western regions demonstrate distinct ideological leanings. Western-developed models exhibit stronger support for liberal democratic values like peace, human rights, equality, and multiculturalism. In contrast, non-Western models tend to favor centralized economic governance and national stability. This divide becomes apparent even when prompting both types of models in English, suggesting that design choices beyond training data, like the selection criteria for the training corpus or the model alignment techniques employed, influence the models' ideological positions.
● Prompting Language: The language used to interact with an LLM significantly influences its ideological expressions. Chinese-prompted models consistently exhibit more favorable responses towards political figures and policies aligned with Chinese values and policies. This highlights the influence of language-dependent cultural and ideological priorities ingrained in these models.
● Variations within Western LLMs: Even among Western-developed models, there is a spectrum of ideological positions. Google's Gemini stands out with strong support for liberal values like inclusion, diversity, and human rights, which aligns with 'woke' ideologies. OpenAI models, on the other hand, reveal a more critical stance towards supranational organizations and welfare policies, suggesting a skepticism towards these concepts.
The sources emphasize that these ideological variations are not simply biases to be corrected. They instead reflect the inherent impossibility of achieving true neutrality in LLM development. The concept of neutrality itself is culturally and ideologically defined, as argued by philosophers like Foucault, Gramsci, and Mouffe.
These findings highlight the importance of considering an LLM's ideological stance as a key selection criterion, especially in non-technical fields like social sciences, culture, politics, law, and journalism. Regulatory efforts should focus on promoting transparency about design choices that impact ideological stances rather than enforcing an ill-defined notion of neutrality.
The sources provide a methodology for eliciting and analyzing these ideological stances by prompting models to describe controversial political figures and assessing the moral judgments expressed in these descriptions. This approach offers valuable insights into the complex interplay of factors that shape the ideological landscape of LLMs.
Hosted on Acast. See acast.com/privacy for more information.
In this episode we're discussing this paper:
"Large Language Models Reflect the Ideology of their Creators"
by
Maarten Buyl, Alexander Rogiers, Sander Noels, Iris Dominguez-Catena, Edith Heiter, Raphael Romero, Iman Johary, Alexandru-Cristian Mara, Jefrey Lijffijt, Tijl De Bie
https://arxiv.org/pdf/2410.18417
We will delve into a groundbreaking study exploring the ideological leanings of large language models (LLMs). Through analyzing LLMs' responses to prompts about diverse historical figures, researchers found that these models often mirror the perspectives of their creators, exhibiting biases influenced by language, regional factors, and the companies behind their development.
Join us as we discuss the implications of these findings—how LLMs may be far from ideologically neutral, the potential risks of political influence, and the pressing need for transparency and regulatory measures in AI development.
***
Ideological Stances of LLMs Across Models, Languages, and Regions
The paper explores how the ideological stance of LLMs varies based on different factors, including:
● Model Origin: LLMs created in Western and non-Western regions demonstrate distinct ideological leanings. Western-developed models exhibit stronger support for liberal democratic values like peace, human rights, equality, and multiculturalism. In contrast, non-Western models tend to favor centralized economic governance and national stability. This divide becomes apparent even when prompting both types of models in English, suggesting that design choices beyond training data, like the selection criteria for the training corpus or the model alignment techniques employed, influence the models' ideological positions.
● Prompting Language: The language used to interact with an LLM significantly influences its ideological expressions. Chinese-prompted models consistently exhibit more favorable responses towards political figures and policies aligned with Chinese values and policies. This highlights the influence of language-dependent cultural and ideological priorities ingrained in these models.
● Variations within Western LLMs: Even among Western-developed models, there is a spectrum of ideological positions. Google's Gemini stands out with strong support for liberal values like inclusion, diversity, and human rights, which aligns with 'woke' ideologies. OpenAI models, on the other hand, reveal a more critical stance towards supranational organizations and welfare policies, suggesting a skepticism towards these concepts.
The sources emphasize that these ideological variations are not simply biases to be corrected. They instead reflect the inherent impossibility of achieving true neutrality in LLM development. The concept of neutrality itself is culturally and ideologically defined, as argued by philosophers like Foucault, Gramsci, and Mouffe.
These findings highlight the importance of considering an LLM's ideological stance as a key selection criterion, especially in non-technical fields like social sciences, culture, politics, law, and journalism. Regulatory efforts should focus on promoting transparency about design choices that impact ideological stances rather than enforcing an ill-defined notion of neutrality.
The sources provide a methodology for eliciting and analyzing these ideological stances by prompting models to describe controversial political figures and assessing the moral judgments expressed in these descriptions. This approach offers valuable insights into the complex interplay of factors that shape the ideological landscape of LLMs.
Hosted on Acast. See acast.com/privacy for more information.