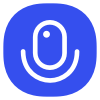
Sign up to save your podcasts
Or
The provided paper, "Chain-of-Thought Matters: Improving Long-Context Language Models with Reasoning Path Supervision," investigates the effectiveness of Chain-of-Thought (CoT) prompting for large language models dealing with long-context tasks, finding that CoT's benefits generally extend and amplify with longer contexts. To enhance performance in these scenarios, the authors introduce LONGREPS, a novel process-supervised framework that trains models to generate high-quality reasoning paths. This framework employs self-sampling of reasoning paths and a specific quality assessment protocol tailored for long contexts, evaluating both answer correctness and process reliability through source faithfulness and intrinsic consistency. Experimental results demonstrate that LONGREPS significantly improves long-context question answering and generalization capabilities compared to standard outcome supervision.
The provided paper, "Chain-of-Thought Matters: Improving Long-Context Language Models with Reasoning Path Supervision," investigates the effectiveness of Chain-of-Thought (CoT) prompting for large language models dealing with long-context tasks, finding that CoT's benefits generally extend and amplify with longer contexts. To enhance performance in these scenarios, the authors introduce LONGREPS, a novel process-supervised framework that trains models to generate high-quality reasoning paths. This framework employs self-sampling of reasoning paths and a specific quality assessment protocol tailored for long contexts, evaluating both answer correctness and process reliability through source faithfulness and intrinsic consistency. Experimental results demonstrate that LONGREPS significantly improves long-context question answering and generalization capabilities compared to standard outcome supervision.