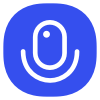
Sign up to save your podcasts
Or
This episode breaks down the 'Lost in the Middle: How Language Models Use Long Contexts' research paper, which investigates how language models use long contexts, specifically examining their ability to access and utilise information placed within the middle of lengthy input sequences. The authors conduct experiments using multi-document question answering and key-value retrieval tasks, finding that performance often degrades when relevant information is not located at the beginning or end of the context. This indicates that current language models struggle to effectively process information distributed throughout their entire context window. The paper then explores potential reasons for this "middle" context weakness, examining factors like model architecture, query-aware contextualization, and instruction fine-tuning. Finally, it concludes with a practical case study of open-domain question answering, demonstrating that language models often fail to leverage additional retrieved documents, highlighting the trade-off between providing more context and the model's ability to effectively process it.
Audio : (Spotify) https://open.spotify.com/episode/4v84xl13Q9aY203SvESyWr?si=fdlPG72GTJKEkyAOwb5RiA
Paper: https://arxiv.org/abs/2307.03172
This episode breaks down the 'Lost in the Middle: How Language Models Use Long Contexts' research paper, which investigates how language models use long contexts, specifically examining their ability to access and utilise information placed within the middle of lengthy input sequences. The authors conduct experiments using multi-document question answering and key-value retrieval tasks, finding that performance often degrades when relevant information is not located at the beginning or end of the context. This indicates that current language models struggle to effectively process information distributed throughout their entire context window. The paper then explores potential reasons for this "middle" context weakness, examining factors like model architecture, query-aware contextualization, and instruction fine-tuning. Finally, it concludes with a practical case study of open-domain question answering, demonstrating that language models often fail to leverage additional retrieved documents, highlighting the trade-off between providing more context and the model's ability to effectively process it.
Audio : (Spotify) https://open.spotify.com/episode/4v84xl13Q9aY203SvESyWr?si=fdlPG72GTJKEkyAOwb5RiA
Paper: https://arxiv.org/abs/2307.03172