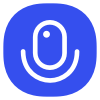
Sign up to save your podcasts
Or
Hey PaperLedge learning crew, Ernis here! Get ready to dive into some fascinating research that tackles a problem we often face when dealing with big, complicated datasets. Think of it like this: you've got a room full of tangled wires (our data), and you need to understand how they're all connected and maybe even simplify the mess to make it manageable.
Researchers have been working on tools to do just that – these are called dimensionality reduction techniques. They help us take data with tons of different characteristics (dimensions) and shrink it down to something we can actually visualize and understand. Think about a photo. It's got millions of pixels (dimensions!). But your brain can easily process that information into a picture of your cat. Dimensionality reduction is kind of like that for any kind of data.
Now, there are already some popular tools out there, like t-SNE and PCA. PCA is like taking a bunch of photos of a building from different angles and then squashing them down into one 2D image that still shows the most important features. It's easy to understand (interpretable), but it can miss some of the more subtle, curvy details (less representational power). T-SNE, on the other hand, can capture those curves and twists, but it's like looking at an abstract painting – you might see something interesting, but it's hard to say exactly why it looks the way it does.
So, here's the problem: we want something that's both powerful and easy to understand. That's where this new paper comes in!
These researchers have created a new algorithm that's like having the best of both worlds. Imagine it like this: instead of just one straight squash (like PCA), they use a series of little squashes, each focused on a different part of the data. These squashes are guided by something called "Gaussian functions," which are like little spotlights that highlight different areas of the data.
The clever thing is that each of these mini-squashes is still simple (linear), so we can understand what it's doing. But by combining them, the algorithm can create really complex and curvy transformations of the data (non-linear). It's like learning to draw a perfect circle by combining a bunch of tiny straight lines. Each line is easy to understand, but together they create something much more sophisticated.
In a nutshell, this new algorithm offers a way to simplify complex data while still letting us see why the simplification works.
The paper also talks about ways to interpret what the algorithm is doing. For instance, it can tell us which dimensions of the original data were squashed the most (suppressed dimensions) and which ones were stretched out (expanded dimensions). This helps us understand what the algorithm thinks is important in the data.
For example, if we're analyzing customer data, maybe the algorithm shows that purchase history is a really important dimension that's been stretched out, while age is less important and has been squashed. That's valuable information for a business!
Why does this matter? Well, for researchers, it gives them a new tool to explore complex datasets in fields like genetics, neuroscience, or even social sciences. For businesses, it could help them better understand their customers, predict market trends, or optimize their operations. And for anyone who's just curious about the world, it's a way to make sense of the massive amounts of data that are constantly being generated.
The researchers even emphasize the importance of creating user-friendly software so that anyone can use this algorithm, not just experts.
So, thinking about this paper, a few things come to mind for our discussion:
That's the gist of it, learning crew! A new way to simplify complex data while keeping the process transparent. I'm excited to hear your thoughts on this one. Until next time, keep exploring!
Hey PaperLedge learning crew, Ernis here! Get ready to dive into some fascinating research that tackles a problem we often face when dealing with big, complicated datasets. Think of it like this: you've got a room full of tangled wires (our data), and you need to understand how they're all connected and maybe even simplify the mess to make it manageable.
Researchers have been working on tools to do just that – these are called dimensionality reduction techniques. They help us take data with tons of different characteristics (dimensions) and shrink it down to something we can actually visualize and understand. Think about a photo. It's got millions of pixels (dimensions!). But your brain can easily process that information into a picture of your cat. Dimensionality reduction is kind of like that for any kind of data.
Now, there are already some popular tools out there, like t-SNE and PCA. PCA is like taking a bunch of photos of a building from different angles and then squashing them down into one 2D image that still shows the most important features. It's easy to understand (interpretable), but it can miss some of the more subtle, curvy details (less representational power). T-SNE, on the other hand, can capture those curves and twists, but it's like looking at an abstract painting – you might see something interesting, but it's hard to say exactly why it looks the way it does.
So, here's the problem: we want something that's both powerful and easy to understand. That's where this new paper comes in!
These researchers have created a new algorithm that's like having the best of both worlds. Imagine it like this: instead of just one straight squash (like PCA), they use a series of little squashes, each focused on a different part of the data. These squashes are guided by something called "Gaussian functions," which are like little spotlights that highlight different areas of the data.
The clever thing is that each of these mini-squashes is still simple (linear), so we can understand what it's doing. But by combining them, the algorithm can create really complex and curvy transformations of the data (non-linear). It's like learning to draw a perfect circle by combining a bunch of tiny straight lines. Each line is easy to understand, but together they create something much more sophisticated.
In a nutshell, this new algorithm offers a way to simplify complex data while still letting us see why the simplification works.
The paper also talks about ways to interpret what the algorithm is doing. For instance, it can tell us which dimensions of the original data were squashed the most (suppressed dimensions) and which ones were stretched out (expanded dimensions). This helps us understand what the algorithm thinks is important in the data.
For example, if we're analyzing customer data, maybe the algorithm shows that purchase history is a really important dimension that's been stretched out, while age is less important and has been squashed. That's valuable information for a business!
Why does this matter? Well, for researchers, it gives them a new tool to explore complex datasets in fields like genetics, neuroscience, or even social sciences. For businesses, it could help them better understand their customers, predict market trends, or optimize their operations. And for anyone who's just curious about the world, it's a way to make sense of the massive amounts of data that are constantly being generated.
The researchers even emphasize the importance of creating user-friendly software so that anyone can use this algorithm, not just experts.
So, thinking about this paper, a few things come to mind for our discussion:
That's the gist of it, learning crew! A new way to simplify complex data while keeping the process transparent. I'm excited to hear your thoughts on this one. Until next time, keep exploring!