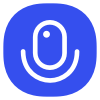
Sign up to save your podcasts
Or
Alright learning crew, Ernis here, ready to dive into some cutting-edge research that could seriously change how we use AI in healthcare! Today, we're tackling a paper about generating synthetic electronic health records, or EHRs. Now, why would we want to fake medical data?
Well, think of it like this: imagine you're trying to train a self-driving car, but you only have footage of driving on sunny days. It'll be great in perfect conditions, but what happens when it starts raining? The car needs to see all sorts of situations to learn properly. The same goes for AI in medicine. We need lots of diverse data to train these models to be truly helpful, but real patient data can be hard to come by due to privacy concerns and simply not having enough examples of rare diseases.
That's where synthetic EHRs come in. They're like computer-generated versions of patient records that can be used to beef up our training datasets. The problem is, most existing methods just try to copy the average patterns they see in real data. It's like teaching our self-driving car to only drive on the most common routes, ignoring those tricky side streets and unexpected obstacles. This means the AI might not be so great at spotting those rare, but super important, medical conditions.
This paper introduces a new approach called TarDiff – short for "Target-Oriented Diffusion". Now, diffusion models are a bit like taking a photo and slowly blurring it until it's just noise, and then reversing the process to bring the image back into focus. TarDiff uses this process to create synthetic EHRs, but with a clever twist. Instead of just blindly recreating the original data's patterns, it focuses on creating data that will specifically help improve the performance of a particular AI model.
Think of it like this: instead of just giving the self-driving car random driving data, we specifically give it data that shows it how to handle icy roads or unexpected deer crossings. TarDiff does this by figuring out how much each synthetic data point is expected to improve the AI's ability to make accurate diagnoses or predictions. It's like having a coach that tells the AI, "Hey, practice this specific scenario, it'll really boost your game!"
So, how does it work in practice? TarDiff uses something called "influence functions" to estimate how much each potential synthetic data point will influence the AI model's performance on a specific task. It then uses this information to guide the diffusion process, making sure it generates data that is most useful for improving the model's accuracy. The researchers tested TarDiff on six different real-world EHR datasets, and the results were pretty impressive. They saw improvements of up to 20.4% in AUPRC (that's a way of measuring how well the AI can identify positive cases) and 18.4% in AUROC (another measure of overall accuracy).
Basically, TarDiff not only creates realistic-looking EHR data, but it also makes sure that the data is actually helpful for training better AI models. This is a big deal because it could help us overcome the challenges of data scarcity and class imbalance, meaning we can train AI to be more effective at diagnosing rare diseases, predicting patient outcomes, and personalizing treatments.
This raises some interesting questions, doesn't it?
Lots to chew on! What do you think learning crew? Let me know your thoughts in the comments!
Alright learning crew, Ernis here, ready to dive into some cutting-edge research that could seriously change how we use AI in healthcare! Today, we're tackling a paper about generating synthetic electronic health records, or EHRs. Now, why would we want to fake medical data?
Well, think of it like this: imagine you're trying to train a self-driving car, but you only have footage of driving on sunny days. It'll be great in perfect conditions, but what happens when it starts raining? The car needs to see all sorts of situations to learn properly. The same goes for AI in medicine. We need lots of diverse data to train these models to be truly helpful, but real patient data can be hard to come by due to privacy concerns and simply not having enough examples of rare diseases.
That's where synthetic EHRs come in. They're like computer-generated versions of patient records that can be used to beef up our training datasets. The problem is, most existing methods just try to copy the average patterns they see in real data. It's like teaching our self-driving car to only drive on the most common routes, ignoring those tricky side streets and unexpected obstacles. This means the AI might not be so great at spotting those rare, but super important, medical conditions.
This paper introduces a new approach called TarDiff – short for "Target-Oriented Diffusion". Now, diffusion models are a bit like taking a photo and slowly blurring it until it's just noise, and then reversing the process to bring the image back into focus. TarDiff uses this process to create synthetic EHRs, but with a clever twist. Instead of just blindly recreating the original data's patterns, it focuses on creating data that will specifically help improve the performance of a particular AI model.
Think of it like this: instead of just giving the self-driving car random driving data, we specifically give it data that shows it how to handle icy roads or unexpected deer crossings. TarDiff does this by figuring out how much each synthetic data point is expected to improve the AI's ability to make accurate diagnoses or predictions. It's like having a coach that tells the AI, "Hey, practice this specific scenario, it'll really boost your game!"
So, how does it work in practice? TarDiff uses something called "influence functions" to estimate how much each potential synthetic data point will influence the AI model's performance on a specific task. It then uses this information to guide the diffusion process, making sure it generates data that is most useful for improving the model's accuracy. The researchers tested TarDiff on six different real-world EHR datasets, and the results were pretty impressive. They saw improvements of up to 20.4% in AUPRC (that's a way of measuring how well the AI can identify positive cases) and 18.4% in AUROC (another measure of overall accuracy).
Basically, TarDiff not only creates realistic-looking EHR data, but it also makes sure that the data is actually helpful for training better AI models. This is a big deal because it could help us overcome the challenges of data scarcity and class imbalance, meaning we can train AI to be more effective at diagnosing rare diseases, predicting patient outcomes, and personalizing treatments.
This raises some interesting questions, doesn't it?
Lots to chew on! What do you think learning crew? Let me know your thoughts in the comments!