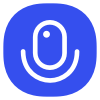
Sign up to save your podcasts
Or
This paper aims to improve reasoning capabilities over long contextual information by learning the relative order of facts and enabling selective attention to its memory. The paper empirically investigates MemReasoner's generalization abilities on multi-hop reasoning tasks compared to other models, even with minimal supervision. Their findings suggest that explicit memory mechanisms can significantly enhance large language models' context processing for reasoning. The authors conclude by discussing limitations, such as the use of synthetic tasks, and suggest future research directions involving more complex real-world scenarios.
This paper aims to improve reasoning capabilities over long contextual information by learning the relative order of facts and enabling selective attention to its memory. The paper empirically investigates MemReasoner's generalization abilities on multi-hop reasoning tasks compared to other models, even with minimal supervision. Their findings suggest that explicit memory mechanisms can significantly enhance large language models' context processing for reasoning. The authors conclude by discussing limitations, such as the use of synthetic tasks, and suggest future research directions involving more complex real-world scenarios.