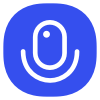
Sign up to save your podcasts
Or
This paper introduces Miras, a novel framework for designing sequence models by drawing parallels between neural architectures and associative memory. The authors reconceptualize models like Transformers and linear RNNs as associative memory modules guided by an "attentional bias" objective. Miras offers a unified perspective based on four key choices: memory architecture, attentional bias, retention gate, and memory learning algorithm. The work presents three new sequence models, Moneta, Yaad, and Memora, developed within this framework, demonstrating enhanced performance in tasks like language modeling and long-context recall compared to existing state-of-the-art models. The framework provides a foundation for understanding and developing future sequence architectures by exploring diverse attentional biases and retention mechanisms. The authors also address making the training of these models more efficient and parallelizable.
This paper introduces Miras, a novel framework for designing sequence models by drawing parallels between neural architectures and associative memory. The authors reconceptualize models like Transformers and linear RNNs as associative memory modules guided by an "attentional bias" objective. Miras offers a unified perspective based on four key choices: memory architecture, attentional bias, retention gate, and memory learning algorithm. The work presents three new sequence models, Moneta, Yaad, and Memora, developed within this framework, demonstrating enhanced performance in tasks like language modeling and long-context recall compared to existing state-of-the-art models. The framework provides a foundation for understanding and developing future sequence architectures by exploring diverse attentional biases and retention mechanisms. The authors also address making the training of these models more efficient and parallelizable.