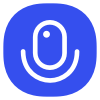
Sign up to save your podcasts
Or
In this episode we break down 'Multi-Scale Context Aggregation by Dilated Convolutions' from Fisher Yu and Vladlen Koltun which investigates the use of dilated convolutions for semantic segmentation in convolutional neural networks. The authors propose a novel context module, which utilises dilated convolutions to aggregate multi-scale contextual information without losing resolution. They demonstrate that this module improves the accuracy of state-of-the-art semantic segmentation architectures on the Pascal VOC 2012 dataset. Furthermore, they analyse the adaptation of image classification networks to dense prediction problems like semantic segmentation, showing that simplifying the adapted network can increase accuracy. The paper also presents experimental results on the CamVid, KITTI, and Cityscapes datasets, demonstrating that the dilated convolution approach outperforms previous methods in urban scene understanding tasks.
Audio : (Spotify) https://open.spotify.com/episode/65E0OXafqV6vOBSkABOd0w?si=CK1xICeoSSeoTK_lBn62Rg
Paper: https://arxiv.org/abs/1511.07122
In this episode we break down 'Multi-Scale Context Aggregation by Dilated Convolutions' from Fisher Yu and Vladlen Koltun which investigates the use of dilated convolutions for semantic segmentation in convolutional neural networks. The authors propose a novel context module, which utilises dilated convolutions to aggregate multi-scale contextual information without losing resolution. They demonstrate that this module improves the accuracy of state-of-the-art semantic segmentation architectures on the Pascal VOC 2012 dataset. Furthermore, they analyse the adaptation of image classification networks to dense prediction problems like semantic segmentation, showing that simplifying the adapted network can increase accuracy. The paper also presents experimental results on the CamVid, KITTI, and Cityscapes datasets, demonstrating that the dilated convolution approach outperforms previous methods in urban scene understanding tasks.
Audio : (Spotify) https://open.spotify.com/episode/65E0OXafqV6vOBSkABOd0w?si=CK1xICeoSSeoTK_lBn62Rg
Paper: https://arxiv.org/abs/1511.07122