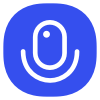
Sign up to save your podcasts
Or
This episode breaks down the 'Neural Machine Translation' paper, which explores a novel approach to neural machine translation, a type of machine translation which employs a single neural network for the translation process. The authors propose an architecture that allows the model to jointly learn to align and translate, overcoming the limitations of previous models that relied on fixed-length vectors to represent entire sentences. By introducing an attention mechanism, the model can focus on the relevant parts of a source sentence while generating each target word, resulting in improved performance, particularly with long sentences. The paper demonstrates that the proposed method achieves translation quality comparable to traditional phrase-based systems, and through qualitative analysis, the authors show that the model's soft-alignments align well with human intuition, suggesting that the approach may have a promising future in natural language processing.
Audio : (Spotify) https://open.spotify.com/episode/5VBNW2nG62fWzn1IHrFiSg?si=oLO1yS-SQOuCCrpiJdS9Iw
Paper: https://arxiv.org/pdf/1409.0473
This episode breaks down the 'Neural Machine Translation' paper, which explores a novel approach to neural machine translation, a type of machine translation which employs a single neural network for the translation process. The authors propose an architecture that allows the model to jointly learn to align and translate, overcoming the limitations of previous models that relied on fixed-length vectors to represent entire sentences. By introducing an attention mechanism, the model can focus on the relevant parts of a source sentence while generating each target word, resulting in improved performance, particularly with long sentences. The paper demonstrates that the proposed method achieves translation quality comparable to traditional phrase-based systems, and through qualitative analysis, the authors show that the model's soft-alignments align well with human intuition, suggesting that the approach may have a promising future in natural language processing.
Audio : (Spotify) https://open.spotify.com/episode/5VBNW2nG62fWzn1IHrFiSg?si=oLO1yS-SQOuCCrpiJdS9Iw
Paper: https://arxiv.org/pdf/1409.0473