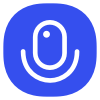
Sign up to save your podcasts
Or
This episode breaks down the 'Neural Message Passing' paper which explores the application of Message Passing Neural Networks (MPNNs) to predict the quantum mechanical properties of molecules. The authors propose a framework that unifies several existing neural network models for graph structured data, enhancing the understanding and creation of novel variations. The paper highlights the state-of-the-art performance of MPNNs on the QM9 dataset, a benchmark of 130,000 molecules with 13 properties each, exceeding the accuracy of traditional Density Functional Theory (DFT) calculations. The authors also investigate the importance of capturing long-range interactions between nodes in the graph and introduce a multi-tower structure to improve scalability and generalization performance. Overall, this work showcases the promise of MPNNs for solving challenging chemical prediction problems, particularly in drug discovery and materials science.
Audio : (Spotify) https://open.spotify.com/episode/0lBjpR4ejpDy7Jwh3Kkn8q?si=3TIklxOlRb2JDwIgDhM5rA
Paper: https://arxiv.org/pdf/1704.01212
This episode breaks down the 'Neural Message Passing' paper which explores the application of Message Passing Neural Networks (MPNNs) to predict the quantum mechanical properties of molecules. The authors propose a framework that unifies several existing neural network models for graph structured data, enhancing the understanding and creation of novel variations. The paper highlights the state-of-the-art performance of MPNNs on the QM9 dataset, a benchmark of 130,000 molecules with 13 properties each, exceeding the accuracy of traditional Density Functional Theory (DFT) calculations. The authors also investigate the importance of capturing long-range interactions between nodes in the graph and introduce a multi-tower structure to improve scalability and generalization performance. Overall, this work showcases the promise of MPNNs for solving challenging chemical prediction problems, particularly in drug discovery and materials science.
Audio : (Spotify) https://open.spotify.com/episode/0lBjpR4ejpDy7Jwh3Kkn8q?si=3TIklxOlRb2JDwIgDhM5rA
Paper: https://arxiv.org/pdf/1704.01212