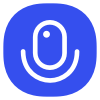
Sign up to save your podcasts
Or
Our guest on the podcast this week is Friederike Schüür, Data Science, Research, and Technical Advisor at Fast Forward Labs.
We discuss how Fast Forward Labs applies the machine learning algorithms of academia to the business world to impact industries. They help clients leverage what they’ve uncovered in their research to build prototypes and demonstrate the potential of new algorithms. Companies are starting to use neural networks for things like image classification, natural language, text summarization, and more. Though it is not a new technique, it is new to the business world and is now available for companies to use. One example of a neural network use-case is to take a long article and automatically cut it down to the five sentences that capture the article’s essence. Artificial Intelligence has been around for more than 30 years, but what has changed now is that it is much easier to store data, and deep learning requires a large amount of data to be effective. Also, the cloud infrastructure makes machine learning possible today because it opens up the use of these algorithms to large companies and even small startups. AI used to require more horsepower, physical space, and cost more to implement than it does today.
Fast Forward Lab clients often struggle to identify the right problem for machine learning. For example, there is a lot of hype around conversational agents, or chat bots, today to replace human agents with AI. But early entrants have found that these bots do not get used frequently by customers, which turns out ot be a user experience problem and not something that can be fixed with machine learning.
The real promise of machine learning is that it can help us with repetitive tasks inside the company. Look in your organization for places where a similar decision or action needs to be made over and over and that is where machine learning could be used.
We discuss the different options for tapping into machine learning algorithms from AWS to Google TensorFlow and open-source tools to combine with them. The most important thing to get started is to determine what clients want to start with defining what the business is trying to achieve. Next it’s important to determine exactly what data is available, and only then to develop a machine learning strategy. Starting simple is important, and only adding complexity when it is necessary.
Our guest on the podcast this week is Friederike Schüür, Data Science, Research, and Technical Advisor at Fast Forward Labs.
We discuss how Fast Forward Labs applies the machine learning algorithms of academia to the business world to impact industries. They help clients leverage what they’ve uncovered in their research to build prototypes and demonstrate the potential of new algorithms. Companies are starting to use neural networks for things like image classification, natural language, text summarization, and more. Though it is not a new technique, it is new to the business world and is now available for companies to use. One example of a neural network use-case is to take a long article and automatically cut it down to the five sentences that capture the article’s essence. Artificial Intelligence has been around for more than 30 years, but what has changed now is that it is much easier to store data, and deep learning requires a large amount of data to be effective. Also, the cloud infrastructure makes machine learning possible today because it opens up the use of these algorithms to large companies and even small startups. AI used to require more horsepower, physical space, and cost more to implement than it does today.
Fast Forward Lab clients often struggle to identify the right problem for machine learning. For example, there is a lot of hype around conversational agents, or chat bots, today to replace human agents with AI. But early entrants have found that these bots do not get used frequently by customers, which turns out ot be a user experience problem and not something that can be fixed with machine learning.
The real promise of machine learning is that it can help us with repetitive tasks inside the company. Look in your organization for places where a similar decision or action needs to be made over and over and that is where machine learning could be used.
We discuss the different options for tapping into machine learning algorithms from AWS to Google TensorFlow and open-source tools to combine with them. The most important thing to get started is to determine what clients want to start with defining what the business is trying to achieve. Next it’s important to determine exactly what data is available, and only then to develop a machine learning strategy. Starting simple is important, and only adding complexity when it is necessary.