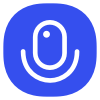
Sign up to save your podcasts
Or
In this episode, we explore a recent legal ruling by the Hamburg Regional Court in the Kneschke v. LAION case, where the court upheld LAION’s data gathering for AI training under EU copyright exemptions for scientific research.
However, the ruling raises concerns about the limitations of current copyright protection mechanisms, particularly the reliance on crawling-stage restrictions like robots.txt.
The discussion introduces a proposed solution: ai.txt, a protocol aimed at verifying rights reservations at the time of model training, addressing gaps in data governance. This episode examines how ai.txt could become a community-driven standard, supporting responsible and equitable AI development.
***
The Hamburg Court's ruling on LAION has significant implications for the future of AI copyright law, particularly concerning the shift in focus from dataset aggregation to the point of model training for copyright enforcement.
● Dataset creation for research purposes, even using copyrighted material, can be permissible under exceptions like Article 3 of the EU Copyright Directive. This was the case with LAION, a non-profit that created a massive dataset of image links for AI training. The court found that their activities fell under the exceptions for scientific research.
● However, the ruling emphasizes that using the dataset to train AI models could still raise copyright infringement concerns. This is because the intended use of the data may not be clear at the data collection stage. Only during model training can one determine if the use falls under exceptions or requires compliance with copyright law.
● The court's distinction between these stages aligns with the AI Act's emphasis on compliance at the model level, as seen in Article 53(1)(c). This suggests that future interpretations of AI copyright issues may concentrate more on the training stage.
● The ruling highlights the limitations of relying solely on crawling-stage restrictions like robots.txt for copyright enforcement. Robots.txt is checked during data collection, but it doesn't account for future data use, which might not be known at that time. Additionally, rights holders' preferences can change, and robots.txt doesn't reflect these updates in already collected datasets.
● The ruling implicitly advocates for time-of-training checks as a more effective mechanism for copyright compliance. This involves verifying data usage rights before feeding it into the AI model during training. This allows for real-time compliance and addresses the evolving nature of AI applications.
● Spawning, a company focused on ethical AI development, proposes ai.txt as a potential solution. It is designed specifically for verifying rights reservations at the time of training. Unlike robots.txt, ai.txt can be applied retroactively and offers more flexibility for expressing permissions. However, ai.txt is envisioned as a community-governed standard that needs input from all stakeholders.
In conclusion, the Hamburg Court's ruling on LAION is a landmark decision that emphasizes the importance of evaluating copyright compliance at the time of AI model training. It underscores the need for new mechanisms like time-of-training checks and protocols like ai.txt to ensure responsible AI development that respects copyright law.
Hosted on Acast. See acast.com/privacy for more information.
In this episode, we explore a recent legal ruling by the Hamburg Regional Court in the Kneschke v. LAION case, where the court upheld LAION’s data gathering for AI training under EU copyright exemptions for scientific research.
However, the ruling raises concerns about the limitations of current copyright protection mechanisms, particularly the reliance on crawling-stage restrictions like robots.txt.
The discussion introduces a proposed solution: ai.txt, a protocol aimed at verifying rights reservations at the time of model training, addressing gaps in data governance. This episode examines how ai.txt could become a community-driven standard, supporting responsible and equitable AI development.
***
The Hamburg Court's ruling on LAION has significant implications for the future of AI copyright law, particularly concerning the shift in focus from dataset aggregation to the point of model training for copyright enforcement.
● Dataset creation for research purposes, even using copyrighted material, can be permissible under exceptions like Article 3 of the EU Copyright Directive. This was the case with LAION, a non-profit that created a massive dataset of image links for AI training. The court found that their activities fell under the exceptions for scientific research.
● However, the ruling emphasizes that using the dataset to train AI models could still raise copyright infringement concerns. This is because the intended use of the data may not be clear at the data collection stage. Only during model training can one determine if the use falls under exceptions or requires compliance with copyright law.
● The court's distinction between these stages aligns with the AI Act's emphasis on compliance at the model level, as seen in Article 53(1)(c). This suggests that future interpretations of AI copyright issues may concentrate more on the training stage.
● The ruling highlights the limitations of relying solely on crawling-stage restrictions like robots.txt for copyright enforcement. Robots.txt is checked during data collection, but it doesn't account for future data use, which might not be known at that time. Additionally, rights holders' preferences can change, and robots.txt doesn't reflect these updates in already collected datasets.
● The ruling implicitly advocates for time-of-training checks as a more effective mechanism for copyright compliance. This involves verifying data usage rights before feeding it into the AI model during training. This allows for real-time compliance and addresses the evolving nature of AI applications.
● Spawning, a company focused on ethical AI development, proposes ai.txt as a potential solution. It is designed specifically for verifying rights reservations at the time of training. Unlike robots.txt, ai.txt can be applied retroactively and offers more flexibility for expressing permissions. However, ai.txt is envisioned as a community-governed standard that needs input from all stakeholders.
In conclusion, the Hamburg Court's ruling on LAION is a landmark decision that emphasizes the importance of evaluating copyright compliance at the time of AI model training. It underscores the need for new mechanisms like time-of-training checks and protocols like ai.txt to ensure responsible AI development that respects copyright law.
Hosted on Acast. See acast.com/privacy for more information.