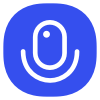
Sign up to save your podcasts
Or
[DISCLAIMER] - For the full visual experience, we recommend you tune in through our YouTube channel to see the presented slides.
If you enjoyed this talk, consider joining the Molecular Modeling and Drug Discovery (M2D2) talks live.
Also consider joining the M2D2 Slack
Abstract: Pre-trained models have been transformative in natural language, computer vision, and now protein sequences by enabling accuracy with few training examples. We show how to use pretrained sequence models in Bayesian optimization to design new protein sequences with minimal labels (i.e., few experiments). Pre-trained models give good predictive accuracy at low data and Bayesian optimization guides the choice of which sequences to test. Pre-trained sequence models also obviate the common requirement of finite pools. Any sequence can be considered. We show significantly fewer labeled sequences are required for many sequence design tasks, including creating novel peptide inhibitors with AlphaFold. This work should enable calibrated predictions with few examples and iterative design with low data (1-50).
Full Paper
Speakers: Ziyue Yang
Twitter Prudencio
Twitter Therence
Twitter Cas
Twitter Valence Discovery
[DISCLAIMER] - For the full visual experience, we recommend you tune in through our YouTube channel to see the presented slides.
If you enjoyed this talk, consider joining the Molecular Modeling and Drug Discovery (M2D2) talks live.
Also consider joining the M2D2 Slack
Abstract: Pre-trained models have been transformative in natural language, computer vision, and now protein sequences by enabling accuracy with few training examples. We show how to use pretrained sequence models in Bayesian optimization to design new protein sequences with minimal labels (i.e., few experiments). Pre-trained models give good predictive accuracy at low data and Bayesian optimization guides the choice of which sequences to test. Pre-trained sequence models also obviate the common requirement of finite pools. Any sequence can be considered. We show significantly fewer labeled sequences are required for many sequence design tasks, including creating novel peptide inhibitors with AlphaFold. This work should enable calibrated predictions with few examples and iterative design with low data (1-50).
Full Paper
Speakers: Ziyue Yang
Twitter Prudencio
Twitter Therence
Twitter Cas
Twitter Valence Discovery