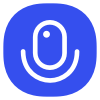
Sign up to save your podcasts
Or
This paper addresses the issue of inefficient tool use by large language models in tool-integrated reasoning. It introduces a novel reinforcement learning framework called Optimal Tool Call-controlled Policy Optimization (OTC-PO). OTC-PO incentivizes models to produce accurate answers while minimizing the number of tool calls. This is achieved through a tool-integrated reward that considers both answer correctness and tool efficiency. Experiments show that OTC-PO significantly reduces tool calls and improves tool productivity without sacrificing accuracy on various question-answering benchmarks. The proposed method offers a way to train more cost-effective and intelligent language agents that can strategically utilize external tools.
This paper addresses the issue of inefficient tool use by large language models in tool-integrated reasoning. It introduces a novel reinforcement learning framework called Optimal Tool Call-controlled Policy Optimization (OTC-PO). OTC-PO incentivizes models to produce accurate answers while minimizing the number of tool calls. This is achieved through a tool-integrated reward that considers both answer correctness and tool efficiency. Experiments show that OTC-PO significantly reduces tool calls and improves tool productivity without sacrificing accuracy on various question-answering benchmarks. The proposed method offers a way to train more cost-effective and intelligent language agents that can strategically utilize external tools.