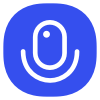
Sign up to save your podcasts
Or
[DISCLAIMER] - For the full visual experience, we recommend you tune in through our YouTube channel to see the presented slides.
If you enjoyed this talk, consider joining the Molecular Modeling and Drug Discovery (M2D2) talks live.
Also, consider joining the M2D2 Slack.
Abstract: Single-cell transcriptomics enabled the study of cellular heterogeneity in response to perturbations at the resolution of individual cells. However, scaling high-throughput screens (HTSs) to measure cellular responses for many drugs remains a challenge due to technical limitations and, more importantly, the cost of such multiplexed experiments. Thus, transferring information from routinely performed bulk RNA-seq HTS is required to enrich single-cell data meaningfully. We introduce a new encoder-decoder architecture to study the perturbational effects of unseen drugs. We combine the model with a transfer learning scheme and demonstrate how training on existing bulk RNA-seq HTS datasets can improve generalisation performance. Better generalisation reduces the need for extensive and costly screens at single-cell resolution. We envision that our proposed method will facilitate more efficient experiment designs through its ability to generate in-silico hypotheses, ultimately accelerating targeted drug discovery.
Full Paper
Speakers: Leon Hetzel and Simon Böhm
Twitter Prudencio
Twitter Therence
Twitter Jonny
Twitter Valence Discovery
[DISCLAIMER] - For the full visual experience, we recommend you tune in through our YouTube channel to see the presented slides.
If you enjoyed this talk, consider joining the Molecular Modeling and Drug Discovery (M2D2) talks live.
Also, consider joining the M2D2 Slack.
Abstract: Single-cell transcriptomics enabled the study of cellular heterogeneity in response to perturbations at the resolution of individual cells. However, scaling high-throughput screens (HTSs) to measure cellular responses for many drugs remains a challenge due to technical limitations and, more importantly, the cost of such multiplexed experiments. Thus, transferring information from routinely performed bulk RNA-seq HTS is required to enrich single-cell data meaningfully. We introduce a new encoder-decoder architecture to study the perturbational effects of unseen drugs. We combine the model with a transfer learning scheme and demonstrate how training on existing bulk RNA-seq HTS datasets can improve generalisation performance. Better generalisation reduces the need for extensive and costly screens at single-cell resolution. We envision that our proposed method will facilitate more efficient experiment designs through its ability to generate in-silico hypotheses, ultimately accelerating targeted drug discovery.
Full Paper
Speakers: Leon Hetzel and Simon Böhm
Twitter Prudencio
Twitter Therence
Twitter Jonny
Twitter Valence Discovery