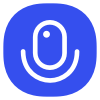
Sign up to save your podcasts
Or
[DISCLAIMER] - For the full visual experience, we recommend you tune in through our YouTube channel to see the presented slides.
If you enjoyed this talk, consider joining the Molecular Modeling and Drug Discovery (M2D2) talks live.
Also, consider joining the M2D2 Slack.
Abstract: The latest biological findings observe that the motionless lock-and-key theory is no longer applicable and that changes in atomic sites and binding pose can provide important information for understanding drug binding. However, the computational expenditure limits the growth of protein trajectory-related studies, thus hindering the possibility of supervised learning. We present a novel spatial-temporal pre-training method based on the modified Equivariant Graph Matching Networks (EGMN), dubbed ProtMD. It has two specially designed self-supervised learning tasks: atom-level prompt-based denoising generative task and conformation-level snapshot ordering task to seize the flexibility information inside MD trajectories with very fine temporal resolutions. More importantly, we investigate the underlying mechanism behind the success of ProtMD, and further demonstrate a tight correlation between the magnitude of spatial motion of conformation and the extent to which the ligand and the receptor bind with each other.
Speaker: Fang Wu
Twitter - Prudencio
Twitter - Therence
Twitter - Jonny
Twitter - Valence Discovery
[DISCLAIMER] - For the full visual experience, we recommend you tune in through our YouTube channel to see the presented slides.
If you enjoyed this talk, consider joining the Molecular Modeling and Drug Discovery (M2D2) talks live.
Also, consider joining the M2D2 Slack.
Abstract: The latest biological findings observe that the motionless lock-and-key theory is no longer applicable and that changes in atomic sites and binding pose can provide important information for understanding drug binding. However, the computational expenditure limits the growth of protein trajectory-related studies, thus hindering the possibility of supervised learning. We present a novel spatial-temporal pre-training method based on the modified Equivariant Graph Matching Networks (EGMN), dubbed ProtMD. It has two specially designed self-supervised learning tasks: atom-level prompt-based denoising generative task and conformation-level snapshot ordering task to seize the flexibility information inside MD trajectories with very fine temporal resolutions. More importantly, we investigate the underlying mechanism behind the success of ProtMD, and further demonstrate a tight correlation between the magnitude of spatial motion of conformation and the extent to which the ligand and the receptor bind with each other.
Speaker: Fang Wu
Twitter - Prudencio
Twitter - Therence
Twitter - Jonny
Twitter - Valence Discovery