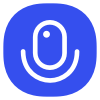
Sign up to save your podcasts
Or
This research paper investigates how to improve the reasoning capabilities of large language models (LLMs), specifically focusing on causal reasoning through counterfactual questions. The authors propose new metrics to better evaluate this reasoning ability and introduce fine-tuning methods that utilize counterfactual feedback to enhance it. Their work also categorizes different ways reasoning can generalize to new problems and evaluates the effectiveness of their fine-tuning approaches across these scenarios, including real-world applications.
This research paper investigates how to improve the reasoning capabilities of large language models (LLMs), specifically focusing on causal reasoning through counterfactual questions. The authors propose new metrics to better evaluate this reasoning ability and introduce fine-tuning methods that utilize counterfactual feedback to enhance it. Their work also categorizes different ways reasoning can generalize to new problems and evaluates the effectiveness of their fine-tuning approaches across these scenarios, including real-world applications.