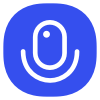
Sign up to save your podcasts
Or
Refined Local Learning Coefficients (rLLCs) are a novel machine learning technique that allows researchers to analyze the specific contributions of individual attention heads within transformer models during training.
This method offers a more detailed understanding of how these heads evolve and contribute to the model's overall performance.
By tracking the development of attention heads over time, rLLCs can pinpoint critical learning phases and potential optimization opportunities within the training process.
This new approach offers a deeper understanding of the inner workings of transformer architectures and has the potential to lead to more efficient training strategies and improved performance in future language models.
Refined Local Learning Coefficients (rLLCs) are a novel machine learning technique that allows researchers to analyze the specific contributions of individual attention heads within transformer models during training.
This method offers a more detailed understanding of how these heads evolve and contribute to the model's overall performance.
By tracking the development of attention heads over time, rLLCs can pinpoint critical learning phases and potential optimization opportunities within the training process.
This new approach offers a deeper understanding of the inner workings of transformer architectures and has the potential to lead to more efficient training strategies and improved performance in future language models.