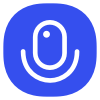
Sign up to save your podcasts
Or
This paper introduces GRADE, a framework for studying teaching effectiveness by examining representational alignment between teachers and students, both human and machine. The authors demonstrate that aligning how a teacher represents information with how a student understands it significantly impacts learning outcomes, even more so than teacher expertise alone. Their findings, supported by simulations and human experiments, show that representational alignment fosters better student accuracy, though this is moderated by classroom size and diversity. Furthermore, the research proposes GRADE-Match, a student-teacher matching procedure that considers alignment and expertise, outperforming standard assignment methods and highlighting the crucial role of shared understanding in effective pedagogy and the design of AI teaching systems.
This paper introduces GRADE, a framework for studying teaching effectiveness by examining representational alignment between teachers and students, both human and machine. The authors demonstrate that aligning how a teacher represents information with how a student understands it significantly impacts learning outcomes, even more so than teacher expertise alone. Their findings, supported by simulations and human experiments, show that representational alignment fosters better student accuracy, though this is moderated by classroom size and diversity. Furthermore, the research proposes GRADE-Match, a student-teacher matching procedure that considers alignment and expertise, outperforming standard assignment methods and highlighting the crucial role of shared understanding in effective pedagogy and the design of AI teaching systems.