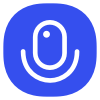
Sign up to save your podcasts
Or
This episode breaks down the 'Retrieval-Augmented Generation for Knowledge-Intensive NLP Tasks' paper, which introduces Retrieval-Augmented Generation (RAG), a new approach to natural language processing (NLP) that combines the strengths of parametric and non-parametric memory. RAG models use a pre-trained language model as a parametric memory to generate text, and a dense vector index of Wikipedia as a non-parametric memory to retrieve relevant information. This approach allows RAG models to access and manipulate factual knowledge more effectively than traditional parametric language models, resulting in improved performance on a variety of knowledge-intensive NLP tasks, including question answering, fact verification, and Jeopardy question generation. The paper demonstrates RAG's ability to update its knowledge by simply replacing its non-parametric memory, making it more adaptable to changing information.
Audio : (Spotify) https://open.spotify.com/episode/13htsegVvyrps0dm9UO08n?si=q5C8iKXrRz2Sdc5ZtWwOEg
Paper: https://arxiv.org/abs/2005.11401v4
This episode breaks down the 'Retrieval-Augmented Generation for Knowledge-Intensive NLP Tasks' paper, which introduces Retrieval-Augmented Generation (RAG), a new approach to natural language processing (NLP) that combines the strengths of parametric and non-parametric memory. RAG models use a pre-trained language model as a parametric memory to generate text, and a dense vector index of Wikipedia as a non-parametric memory to retrieve relevant information. This approach allows RAG models to access and manipulate factual knowledge more effectively than traditional parametric language models, resulting in improved performance on a variety of knowledge-intensive NLP tasks, including question answering, fact verification, and Jeopardy question generation. The paper demonstrates RAG's ability to update its knowledge by simply replacing its non-parametric memory, making it more adaptable to changing information.
Audio : (Spotify) https://open.spotify.com/episode/13htsegVvyrps0dm9UO08n?si=q5C8iKXrRz2Sdc5ZtWwOEg
Paper: https://arxiv.org/abs/2005.11401v4