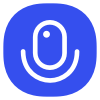
Sign up to save your podcasts
Or
Google DeepMind researchers have developed a new robotic task learning method called RT-Affordance, which uses affordances as a bridge between high-level planning and low-level action execution.
This method breaks down complex tasks into simpler sub-tasks, making robots more adaptable to different environments and tasks.
RT-Affordance consists of a planner, an affordance detector, and a controller, enabling robots to understand the possibilities of action within their environment and execute actions accordingly.
This innovative approach contributes to the advancement of more flexible and capable robotic systems for real-world applications.
Google DeepMind researchers have developed a new robotic task learning method called RT-Affordance, which uses affordances as a bridge between high-level planning and low-level action execution.
This method breaks down complex tasks into simpler sub-tasks, making robots more adaptable to different environments and tasks.
RT-Affordance consists of a planner, an affordance detector, and a controller, enabling robots to understand the possibilities of action within their environment and execute actions accordingly.
This innovative approach contributes to the advancement of more flexible and capable robotic systems for real-world applications.