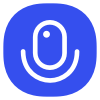
Sign up to save your podcasts
Or
This research paper introduces QALIGN, a novel test-time method to enhance language model outputs by sampling from a more optimal distribution without requiring model retraining or even access to internal model details. Existing test-time compute methods that rely on reward models for selection can degrade with increased computation due to over-optimization of these imperfect proxies. QALIGN, leveraging Markov chain Monte Carlo techniques, refines outputs on a per-prompt basis as more computation is applied, leading to consistently better-aligned results on mathematical reasoning and general knowledge benchmarks compared to methods like best-of-n and majority voting, and even outperforming models fine-tuned with direct preference optimization. This approach offers a practical way to improve off-the-shelf language model capabilities at inference time, especially when model weights are inaccessible.
This research paper introduces QALIGN, a novel test-time method to enhance language model outputs by sampling from a more optimal distribution without requiring model retraining or even access to internal model details. Existing test-time compute methods that rely on reward models for selection can degrade with increased computation due to over-optimization of these imperfect proxies. QALIGN, leveraging Markov chain Monte Carlo techniques, refines outputs on a per-prompt basis as more computation is applied, leading to consistently better-aligned results on mathematical reasoning and general knowledge benchmarks compared to methods like best-of-n and majority voting, and even outperforming models fine-tuned with direct preference optimization. This approach offers a practical way to improve off-the-shelf language model capabilities at inference time, especially when model weights are inaccessible.