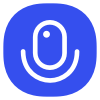
Sign up to save your podcasts
Or
This research paper proposes a new method for achieving sparsity in attention models, called Rectified Linear Attention (ReLA). ReLA replaces the softmax function with a ReLU activation, leading to sparsity by dropping negative attention scores. To stabilise gradient training, layer normalisation with a specialized initialization or gating mechanism is used. Experiments on five machine translation tasks show that ReLA achieves comparable translation performance to softmax-based models, while being more efficient than other sparse attention mechanisms. The authors also conduct in-depth analysis of ReLA's performance, finding that it exhibits high sparsity, head diversity, and aligns better with word alignment than other methods. Furthermore, ReLA has the intriguing ability to "switch off" attention heads for some queries, allowing for highly specialized heads and potentially indicating translation quality.
This research paper proposes a new method for achieving sparsity in attention models, called Rectified Linear Attention (ReLA). ReLA replaces the softmax function with a ReLU activation, leading to sparsity by dropping negative attention scores. To stabilise gradient training, layer normalisation with a specialized initialization or gating mechanism is used. Experiments on five machine translation tasks show that ReLA achieves comparable translation performance to softmax-based models, while being more efficient than other sparse attention mechanisms. The authors also conduct in-depth analysis of ReLA's performance, finding that it exhibits high sparsity, head diversity, and aligns better with word alignment than other methods. Furthermore, ReLA has the intriguing ability to "switch off" attention heads for some queries, allowing for highly specialized heads and potentially indicating translation quality.