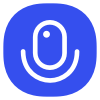
Sign up to save your podcasts
Or
DeepSeek has taken the AI world by storm, sparking excitement, skepticism, and heated debates. Is this the next big leap in AI reasoning, or is it just another overhyped model? In this episode, we peel back the layers of DeepSeek-R1 and DeepSeek-V3, diving into the technology behind its Mixture of Experts (MoE), Multi-Head Latent Attention (MLA), Multi-Token Prediction (MTP), and Reinforcement Learning (GRPO) approaches. We also take a hard look at the training costs—is it really just $5.6M, or is the actual number closer to $80M-$100M?
Join us as we break down:
At the end of the episode, we answer the big question: DeepSeek – WOW or MEH?
Key Topics Discussed:
References & Further Reading:
DeepSeek has taken the AI world by storm, sparking excitement, skepticism, and heated debates. Is this the next big leap in AI reasoning, or is it just another overhyped model? In this episode, we peel back the layers of DeepSeek-R1 and DeepSeek-V3, diving into the technology behind its Mixture of Experts (MoE), Multi-Head Latent Attention (MLA), Multi-Token Prediction (MTP), and Reinforcement Learning (GRPO) approaches. We also take a hard look at the training costs—is it really just $5.6M, or is the actual number closer to $80M-$100M?
Join us as we break down:
At the end of the episode, we answer the big question: DeepSeek – WOW or MEH?
Key Topics Discussed:
References & Further Reading: