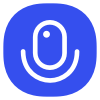
Sign up to save your podcasts
Or
In this must-listen episode of the Enterprise GTM Podcast, I dive deep with two powerhouse AI experts:
🎙 Manasi Vartak (Chief AI Architect at Cloudera)
🎙 Shreya Shankar (PhD Candidate, UC Berkeley)
We discuss the current landscape of GenAI in enterprises. We explore the challenges of productionizing AI applications, the importance of data privacy, and the evolving use cases within large organizations. Manasi shares insights from her experience at Cloudera, highlighting the slow adoption of GenAI in production and the prevalent use of internal applications. Shreya elaborates on her research at UC Berkeley, including how her work could transform data engineering.
The conversation emphasizes the need for new tools and strategies to navigate the complexities of AI deployment and the significance of benchmarking in this evolving field. This conversation explores the evolving landscape of data engineering and the role of Generative AI (Gen.AI) in automating processes, simplifying data management, and enhancing user experience.
The speakers discuss the importance of coding assistants, the need for new benchmarks in AI tools, and the critical role of user experience in designing AI applications. They emphasize the necessity of education and training to help users effectively leverage AI technologies in their workflows.
Takeaways
* The excitement about GenAI remains strong in enterprises.
* Adoption of GenAI in production is still under 10%.
* Internal use cases dominate the current landscape of GenAI applications.
* The RAG pattern is a leading use case for enterprises.
* Data privacy and security are major concerns for enterprises.
* AI is becoming more democratized, allowing non-experts to build applications.
* Productionizing AI requires significant effort and infrastructure.
* Shreya's research focuses on optimizing database interactions with GenAI.
* Accuracy in prompting is a complex and fuzzy concept.
* Benchmarking in GenAI is challenging due to the lack of ground truth. The future of data engineering will involve more automation and less manual toil.
* Gen.AI can simplify data management tasks significantly.
* Natural language processing will make data access easier for non-technical users.
* User experience is crucial for the success of AI applications.
* AI tools should be designed for seamless human-AI collaboration.
* Benchmarks for AI tools need to be redefined beyond traditional accuracy measures.
* Education is key to helping users effectively utilize AI technologies.
* Domain experts will benefit from tailored coding assistants in their specific environments.
* The transition from creation to review in AI applications is essential for user engagement.
* Accessibility of Gen.AI presents new opportunities for education and innovation.
Chapters
00:00 Introduction to AI Experts
02:05 Current State of GenAI in Enterprises
05:58 Use Cases and Adoption Challenges
11:59 Parallels in AI Evolution
16:02 Shreya's Research on GenAI and Databases
22:41 The Future of Data Engineering and Automation
24:11 Simplifying Data Management with Gen.AI
27:20 The Role of Coding Assistants in Data Tasks
30:47 Rethinking Benchmarks for AI Tools
35:33 User Experience in AI Applications
39:20 Designing for Human-AI Collaboration
44:18 Education and Training for Effective AI Use
Links mentioned in today’s episode:
Shreya Shankar
Shreya’s work DocETL
Manasi Vartak on Linkedin
In this must-listen episode of the Enterprise GTM Podcast, I dive deep with two powerhouse AI experts:
🎙 Manasi Vartak (Chief AI Architect at Cloudera)
🎙 Shreya Shankar (PhD Candidate, UC Berkeley)
We discuss the current landscape of GenAI in enterprises. We explore the challenges of productionizing AI applications, the importance of data privacy, and the evolving use cases within large organizations. Manasi shares insights from her experience at Cloudera, highlighting the slow adoption of GenAI in production and the prevalent use of internal applications. Shreya elaborates on her research at UC Berkeley, including how her work could transform data engineering.
The conversation emphasizes the need for new tools and strategies to navigate the complexities of AI deployment and the significance of benchmarking in this evolving field. This conversation explores the evolving landscape of data engineering and the role of Generative AI (Gen.AI) in automating processes, simplifying data management, and enhancing user experience.
The speakers discuss the importance of coding assistants, the need for new benchmarks in AI tools, and the critical role of user experience in designing AI applications. They emphasize the necessity of education and training to help users effectively leverage AI technologies in their workflows.
Takeaways
* The excitement about GenAI remains strong in enterprises.
* Adoption of GenAI in production is still under 10%.
* Internal use cases dominate the current landscape of GenAI applications.
* The RAG pattern is a leading use case for enterprises.
* Data privacy and security are major concerns for enterprises.
* AI is becoming more democratized, allowing non-experts to build applications.
* Productionizing AI requires significant effort and infrastructure.
* Shreya's research focuses on optimizing database interactions with GenAI.
* Accuracy in prompting is a complex and fuzzy concept.
* Benchmarking in GenAI is challenging due to the lack of ground truth. The future of data engineering will involve more automation and less manual toil.
* Gen.AI can simplify data management tasks significantly.
* Natural language processing will make data access easier for non-technical users.
* User experience is crucial for the success of AI applications.
* AI tools should be designed for seamless human-AI collaboration.
* Benchmarks for AI tools need to be redefined beyond traditional accuracy measures.
* Education is key to helping users effectively utilize AI technologies.
* Domain experts will benefit from tailored coding assistants in their specific environments.
* The transition from creation to review in AI applications is essential for user engagement.
* Accessibility of Gen.AI presents new opportunities for education and innovation.
Chapters
00:00 Introduction to AI Experts
02:05 Current State of GenAI in Enterprises
05:58 Use Cases and Adoption Challenges
11:59 Parallels in AI Evolution
16:02 Shreya's Research on GenAI and Databases
22:41 The Future of Data Engineering and Automation
24:11 Simplifying Data Management with Gen.AI
27:20 The Role of Coding Assistants in Data Tasks
30:47 Rethinking Benchmarks for AI Tools
35:33 User Experience in AI Applications
39:20 Designing for Human-AI Collaboration
44:18 Education and Training for Effective AI Use
Links mentioned in today’s episode:
Shreya Shankar
Shreya’s work DocETL
Manasi Vartak on Linkedin