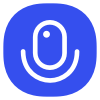
Sign up to save your podcasts
Or
Gwern.net's "The Scaling Hypothesis" explores the idea that artificial general intelligence (AGI) can emerge simply by scaling up neural networks with more data and compute. It centers on GPT-3 as a demonstration of "blessings of scale," where larger models exhibit meta-learning and surprising capabilities. The text challenges the views of AI researchers who downplay the potential of scaling and argues that current approaches may already be on a path to AGI. Furthermore, the author suggests that agency, traditionally viewed as a discrete property, is in fact a continuum and thus may unexpectedly arise in more AI models than people think. It also examines the reasons for the skepticism surrounding the scaling hypothesis, even in the face of compelling results. Lastly, the document includes excerpts from "GPT-3: Language Models are Few-Shot Learners", Brown et al 2020.
Link to work: https://gwern.net/scaling-hypothesis
Hosted on Acast. See acast.com/privacy for more information.
Gwern.net's "The Scaling Hypothesis" explores the idea that artificial general intelligence (AGI) can emerge simply by scaling up neural networks with more data and compute. It centers on GPT-3 as a demonstration of "blessings of scale," where larger models exhibit meta-learning and surprising capabilities. The text challenges the views of AI researchers who downplay the potential of scaling and argues that current approaches may already be on a path to AGI. Furthermore, the author suggests that agency, traditionally viewed as a discrete property, is in fact a continuum and thus may unexpectedly arise in more AI models than people think. It also examines the reasons for the skepticism surrounding the scaling hypothesis, even in the face of compelling results. Lastly, the document includes excerpts from "GPT-3: Language Models are Few-Shot Learners", Brown et al 2020.
Link to work: https://gwern.net/scaling-hypothesis
Hosted on Acast. See acast.com/privacy for more information.