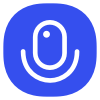
Sign up to save your podcasts
Or
This paper mathematically models the scheduling of Large Language Model (LLM) inference tasks, a growing area of computational demand. It introduces a queuing theory framework to analyze and optimize the throughput of LLM serving systems, considering the distinct prefill and decode phases of processing. The authors identify conditions under which work-conserving scheduling algorithms can achieve maximum throughput for single LLM instances and explore the complexities introduced by AI agent workloads involving multiple interacting LLMs. They also examine the practical impact of scheduling choices, such as token budget, on latency performance and discuss the limitations of certain existing scheduling approaches. The work provides a theoretical foundation for understanding and improving the efficiency of LLM inference and multi-agent AI systems.
This paper mathematically models the scheduling of Large Language Model (LLM) inference tasks, a growing area of computational demand. It introduces a queuing theory framework to analyze and optimize the throughput of LLM serving systems, considering the distinct prefill and decode phases of processing. The authors identify conditions under which work-conserving scheduling algorithms can achieve maximum throughput for single LLM instances and explore the complexities introduced by AI agent workloads involving multiple interacting LLMs. They also examine the practical impact of scheduling choices, such as token budget, on latency performance and discuss the limitations of certain existing scheduling approaches. The work provides a theoretical foundation for understanding and improving the efficiency of LLM inference and multi-agent AI systems.