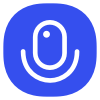
Sign up to save your podcasts
Or
[DISCLAIMER] - For the full visual experience, we recommend you tune in through our YouTube channel to see the presented slides.
Try datamol.io - the open source toolkit that simplifies molecular processing and featurization workflows for machine learning scientists working in drug discovery: https://datamol.io/
If you enjoyed this talk, consider joining the Molecular Modeling and Drug Discovery (M2D2) talks live.
Also, consider joining the M2D2 Slack.
Abstract: Cryptic pockets, which are absent in ligand-free structures and have the potential to be used as drug targets, are often challenging to access through conventional biomolecular simulations due to their slow motions. To overcome this limitation, we have combined AlphaFold and Markov State modelling (MSM) to accelerate the discovery of cryptic pockets. AlphaFold was used to generate a diverse structural ensemble with open or partially open pockets that can serve as starting points for molecular dynamics simulations which were later stitched together using MSM to predict free energy and kinetics associated with cryptic pocket opening. Our approach explored known cryptic pockets, as well as discovered new cryptic pockets which were absent in PDB. Our study highlighted the power of AlphaFold and MSM to discover novel cryptic pockets which can unlock development of next-gen therapeutics.
Speaker: Stephan Thaler
Twitter - Prudencio
Twitter - Jonny
Twitter - datamol.io
[DISCLAIMER] - For the full visual experience, we recommend you tune in through our YouTube channel to see the presented slides.
Try datamol.io - the open source toolkit that simplifies molecular processing and featurization workflows for machine learning scientists working in drug discovery: https://datamol.io/
If you enjoyed this talk, consider joining the Molecular Modeling and Drug Discovery (M2D2) talks live.
Also, consider joining the M2D2 Slack.
Abstract: Cryptic pockets, which are absent in ligand-free structures and have the potential to be used as drug targets, are often challenging to access through conventional biomolecular simulations due to their slow motions. To overcome this limitation, we have combined AlphaFold and Markov State modelling (MSM) to accelerate the discovery of cryptic pockets. AlphaFold was used to generate a diverse structural ensemble with open or partially open pockets that can serve as starting points for molecular dynamics simulations which were later stitched together using MSM to predict free energy and kinetics associated with cryptic pocket opening. Our approach explored known cryptic pockets, as well as discovered new cryptic pockets which were absent in PDB. Our study highlighted the power of AlphaFold and MSM to discover novel cryptic pockets which can unlock development of next-gen therapeutics.
Speaker: Stephan Thaler
Twitter - Prudencio
Twitter - Jonny
Twitter - datamol.io