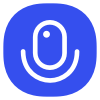
Sign up to save your podcasts
Or
The provided text introduces VCR-Bench, a novel benchmark designed to evaluate the Chain-of-Thought (CoT) reasoning capabilities of large vision-language models (LVLMs) in video understanding. Current benchmarks for video understanding often fall short by not thoroughly assessing the reasoning process, focusing mainly on final answer accuracy and struggling to differentiate between perception and reasoning abilities. To address these limitations, VCR-Bench offers a multi-dimensional evaluation framework with detailed annotations of reasoning steps across diverse video types and tasks. Evaluations using VCR-Bench reveal that current LVLMs still have significant shortcomings in video reasoning, particularly in extracting and understanding temporal-spatial information, despite a strong correlation between CoT quality and answer accuracy.
The provided text introduces VCR-Bench, a novel benchmark designed to evaluate the Chain-of-Thought (CoT) reasoning capabilities of large vision-language models (LVLMs) in video understanding. Current benchmarks for video understanding often fall short by not thoroughly assessing the reasoning process, focusing mainly on final answer accuracy and struggling to differentiate between perception and reasoning abilities. To address these limitations, VCR-Bench offers a multi-dimensional evaluation framework with detailed annotations of reasoning steps across diverse video types and tasks. Evaluations using VCR-Bench reveal that current LVLMs still have significant shortcomings in video reasoning, particularly in extracting and understanding temporal-spatial information, despite a strong correlation between CoT quality and answer accuracy.