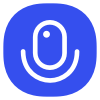
Sign up to save your podcasts
Or
Recent progress in video generation still struggles with issues like motion instability and prompt alignment. To address this, the study explores incorporating human preferences into advanced flow-based video generation models. The authors introduce a large, new dataset of human-annotated video preferences across visual quality, motion quality, and text alignment. They also develop a multi-dimensional reward model to quantify these preferences and propose three alignment algorithms for flow-based models, demonstrating that a modified Direct Preference Optimization method yields the most effective results in aligning video generation with human expectations.
Recent progress in video generation still struggles with issues like motion instability and prompt alignment. To address this, the study explores incorporating human preferences into advanced flow-based video generation models. The authors introduce a large, new dataset of human-annotated video preferences across visual quality, motion quality, and text alignment. They also develop a multi-dimensional reward model to quantify these preferences and propose three alignment algorithms for flow-based models, demonstrating that a modified Direct Preference Optimization method yields the most effective results in aligning video generation with human expectations.