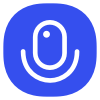
Sign up to save your podcasts
Or
This paper introduces CoT-VLA, a novel method for vision-language-action models (VLAs) that incorporates visual chain-of-thought (CoT) reasoning. Unlike traditional VLAs that directly map inputs to actions, CoT-VLA first predicts future image frames as visual goals before generating action sequences to achieve them. This approach aims to enhance reasoning capabilities for complex manipulation tasks by leveraging both robot demonstrations and unlabeled video data. The paper details the model's architecture, training procedures, and experimental results demonstrating improved performance on simulated and real-world robotic tasks compared to existing VLA methods.
This paper introduces CoT-VLA, a novel method for vision-language-action models (VLAs) that incorporates visual chain-of-thought (CoT) reasoning. Unlike traditional VLAs that directly map inputs to actions, CoT-VLA first predicts future image frames as visual goals before generating action sequences to achieve them. This approach aims to enhance reasoning capabilities for complex manipulation tasks by leveraging both robot demonstrations and unlabeled video data. The paper details the model's architecture, training procedures, and experimental results demonstrating improved performance on simulated and real-world robotic tasks compared to existing VLA methods.