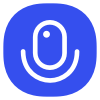
Sign up to save your podcasts
Or
This paper challenges the traditional view that reward model accuracy is the sole determinant of success in Reinforcement Learning from Human Feedback (RLHF). It posits from an optimization perspective that while accuracy reflects alignment with ground truth, a critical factor often overlooked is reward variance, which influences the RLHF objective landscape. The authors demonstrate theoretically and empirically that low reward variance can lead to a flat optimization landscape, causing even highly accurate reward models to be less effective teachers than less accurate ones that induce sufficient variance. Furthermore, the study reveals that a reward model's effectiveness is not universal, as the same model can perform differently for various language models due to variations in induced reward variance. This highlights the limitations of evaluating reward models solely based on accuracy or in isolation from the language model they are intended to guide.
This paper challenges the traditional view that reward model accuracy is the sole determinant of success in Reinforcement Learning from Human Feedback (RLHF). It posits from an optimization perspective that while accuracy reflects alignment with ground truth, a critical factor often overlooked is reward variance, which influences the RLHF objective landscape. The authors demonstrate theoretically and empirically that low reward variance can lead to a flat optimization landscape, causing even highly accurate reward models to be less effective teachers than less accurate ones that induce sufficient variance. Furthermore, the study reveals that a reward model's effectiveness is not universal, as the same model can perform differently for various language models due to variations in induced reward variance. This highlights the limitations of evaluating reward models solely based on accuracy or in isolation from the language model they are intended to guide.