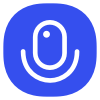
Sign up to save your podcasts
Or
This introduces WikiBigEdit, a new large-scale benchmark for evaluating how well large language models can continuously update their factual knowledge over time, using real-world edits from Wikidata. The authors find that existing knowledge editing techniques struggle with the scale and sequential nature of these real-world updates. In contrast, simpler methods like retrieval augmentation and continual finetuning with model merging prove more effective for incorporating and retaining a large volume of evolving information. Ultimately, the work highlights the limitations of current knowledge editing approaches at practical scales and suggests that more standard techniques offer promising alternatives for keeping language models factually current.
This introduces WikiBigEdit, a new large-scale benchmark for evaluating how well large language models can continuously update their factual knowledge over time, using real-world edits from Wikidata. The authors find that existing knowledge editing techniques struggle with the scale and sequential nature of these real-world updates. In contrast, simpler methods like retrieval augmentation and continual finetuning with model merging prove more effective for incorporating and retaining a large volume of evolving information. Ultimately, the work highlights the limitations of current knowledge editing approaches at practical scales and suggests that more standard techniques offer promising alternatives for keeping language models factually current.