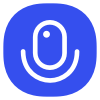
Sign up to save your podcasts
Or
This episode breaks down the 'Zephyr: Direct Distillation of LM Alignment' research paper, which describes ZEPHYR-7B, a smaller language model (LLM) aligned with user intent, which outperforms larger LLMs on chat benchmarks despite being trained using only distilled supervised fine-tuning (dSFT) and distilled direct preference optimisation (dDPO). The paper outlines three main steps in the development of this model: dSFT, where the model is fine-tuned using outputs from a larger teacher model; AI Feedback (AIF), where the teacher model ranks responses from other models; and dDPO, which uses the preference data collected in AIF to further refine the model. The paper then compares the performance of ZEPHYR-7B to other open-source and proprietary LLMs, demonstrating the effectiveness of its approach.
Audio : (Spotify) https://open.spotify.com/episode/0TrFFR6dXgbdU2SZLo5k0j?si=wkhUBTGlSJKnUsPBwYY3-w
Paper: https://arxiv.org/pdf/2310.16944.pdf
This episode breaks down the 'Zephyr: Direct Distillation of LM Alignment' research paper, which describes ZEPHYR-7B, a smaller language model (LLM) aligned with user intent, which outperforms larger LLMs on chat benchmarks despite being trained using only distilled supervised fine-tuning (dSFT) and distilled direct preference optimisation (dDPO). The paper outlines three main steps in the development of this model: dSFT, where the model is fine-tuned using outputs from a larger teacher model; AI Feedback (AIF), where the teacher model ranks responses from other models; and dDPO, which uses the preference data collected in AIF to further refine the model. The paper then compares the performance of ZEPHYR-7B to other open-source and proprietary LLMs, demonstrating the effectiveness of its approach.
Audio : (Spotify) https://open.spotify.com/episode/0TrFFR6dXgbdU2SZLo5k0j?si=wkhUBTGlSJKnUsPBwYY3-w
Paper: https://arxiv.org/pdf/2310.16944.pdf