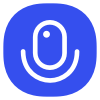
Sign up to save your podcasts
Or
Today I am talking to Philip Winter, researcher at the Medical Imaging group of the VRVis, a research center for virtual realities and visualizations.
Philip will explain the benefits and challenges in continual learning and will present his recent paper "PARMESAN: Parameter-Free Memory Search and Transduction for Dense Prediction Tasks". Where he and his colleagues have developed a system that uses a frozen hierarchical feature extractor to build a memory database out of the labeled training data. During inference the system identified training examples similar to the test data and prediction is performed through a combination of parameter-free correspondence matching and message passing based on the closes training datapoints.
I hope you enjoy this episode and will find it useful.
## AAIP Community
Join our discord server and ask guest directly or discuss related topics with the community.
https://discord.gg/5Pj446VKNU
## TOC
00:00:00 Beginning
00:03:04 Guest Introduction
00:06:50 What is continual learning?
00:15:38 Catastrophic forgetting
00:27:36 Paper: Parmesan
00:40:14 Composing Ensembles
00:46:12 How to build memory over time
00:55:37 Limitations of Parmesan
### References
Philip Winter - https://www.linkedin.com/in/philip-m-winter-msc-b15679129/
VRVIS - https://www.vrvis.at/
PARMESAN: Parameter-Free Memory Search and Transduction for Dense Prediction Tasks - https://arxiv.org/abs/2403.11743
Continual Learning Survey: https://arxiv.org/pdf/1909.08383
Today I am talking to Philip Winter, researcher at the Medical Imaging group of the VRVis, a research center for virtual realities and visualizations.
Philip will explain the benefits and challenges in continual learning and will present his recent paper "PARMESAN: Parameter-Free Memory Search and Transduction for Dense Prediction Tasks". Where he and his colleagues have developed a system that uses a frozen hierarchical feature extractor to build a memory database out of the labeled training data. During inference the system identified training examples similar to the test data and prediction is performed through a combination of parameter-free correspondence matching and message passing based on the closes training datapoints.
I hope you enjoy this episode and will find it useful.
## AAIP Community
Join our discord server and ask guest directly or discuss related topics with the community.
https://discord.gg/5Pj446VKNU
## TOC
00:00:00 Beginning
00:03:04 Guest Introduction
00:06:50 What is continual learning?
00:15:38 Catastrophic forgetting
00:27:36 Paper: Parmesan
00:40:14 Composing Ensembles
00:46:12 How to build memory over time
00:55:37 Limitations of Parmesan
### References
Philip Winter - https://www.linkedin.com/in/philip-m-winter-msc-b15679129/
VRVIS - https://www.vrvis.at/
PARMESAN: Parameter-Free Memory Search and Transduction for Dense Prediction Tasks - https://arxiv.org/abs/2403.11743
Continual Learning Survey: https://arxiv.org/pdf/1909.08383
12 Listeners